Deepfakes have become a growing concern in recent years as technological advancements have made it easier to manipulate digital media. These artificial intelligence-generated videos, images, and audio files have the potential to deceive people and spread disinformation on an unprecedented scale. In this article, we explore the evolution of deepfakes, how they work, and how to identify them.
What is a deepfake?
Deepfakes, a combination of the words “deep learning” and “fake,” refer to manipulated digital media that replace the likeness of one person with that of another convincingly. It involves training a machine learning model, such as a neural network, to generate images, videos, or audio that mimic the appearance or sound of a particular person or object.
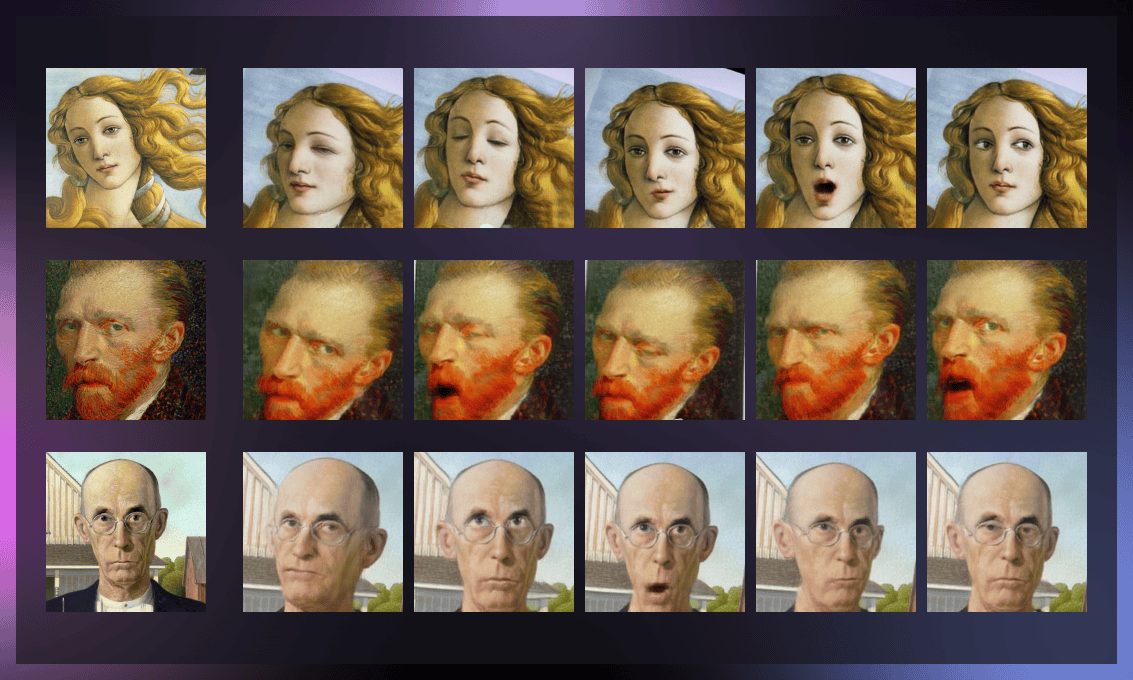
What are some examples of deepfakes?
Recently, the Republican National Committee released a 30-second video imagining what President Joe Biden’s second term might look like (nothing but catastrophic).
Several years ago, a Reddit user, “deepfakes,” launched a forum with pornographic content created by superimposing actors’ faces in videos with faces of other people. This type of fakes has garnered significant media attention and severely damaged the reputations of public figures and celebrities.
A few months later, to draw attention to the potentially disruptive technology, BuzzFeed published a video that appeared to depict former US President Barack Obama using foul language and hurling insults at President Donald Trump. The media outlet disclosed that the video had been manipulated to create a deepfake. The altered clip featured the voice of actor and director Jordan Peele, which was integrated into the original Obama footage.
In 2019, a video, in which Nancy Pelosi, the Speaker of the US House of Representatives, appeared to be drunkenly slurring through a speech, went viral on Facebook and YouTube. The doctored video was reported to be first posted on Facebook by the sports blogger Shawn Brooks from New York.
Although deepfakes have negative aspects, they find good applications as well, specifically in art and entertainment. Deepfakes can generate entirely new images, which can be a valuable tool for artists. This technology enables users to animate still pictures, produce videos of people and events that cannot be captured in real life, and embody characters or other individuals. For instance, In 2018, photos and videos of a young Harrison Ford from 1977 were used to create a convincing depiction of a youthful Han Solo in “Solo: A Star Wars Story.”
Another example is a humorous deepfake created by Colider featuring the faces of Tom Cruise, Robert Downey Jr., George Lucas, Ewan McGregor, and Jeff Goldblum discussing the future of cinema and streaming.
Deepfakes have also been used to enhance museum and exhibition experience.
At the 2019 show at The Dali Museum in St. Petersburg, Florida, visitors were able to engage with a lifelike of the artist featured on a series of screens.
British artist Gillian Wearing, whose art practice focuses on themes of human identity and experience, used deepfakes to make the short film “Wearing Gillian” (2018). In her conceptual work, the artist uses makeup, costumes, and masks to embody iconic figures like Georgia O’Keeffe and Albrecht Durer. However, she takes a different route in the film by superimposing her face onto the actors who portray her. This application of deepfake technology challenges viewers’ perception of reality and identity. Wearing opens up about her sense of unease and detachment as she watches herself portrayed on screen by someone else.
How do deepfakes work?
Fake content is generated and refined through two generative adversarial networks (GANs): the generator and the discriminator. These are special types of deep learning models used for generating new data based on the samples provided.
The generator is fed a random noise vector as input to create a synthetic image that is then presented to the discriminator alongside real images. Through examining the distinguishing features of real images, the discriminator learns to differentiate between real and fake ones, while the generator seeks to create more realistic images to deceive the discriminator. As training continues, both the generator and discriminator improve their respective abilities until the generator produces images that are indistinguishable from real ones, or the discriminator fails to differentiate between them.
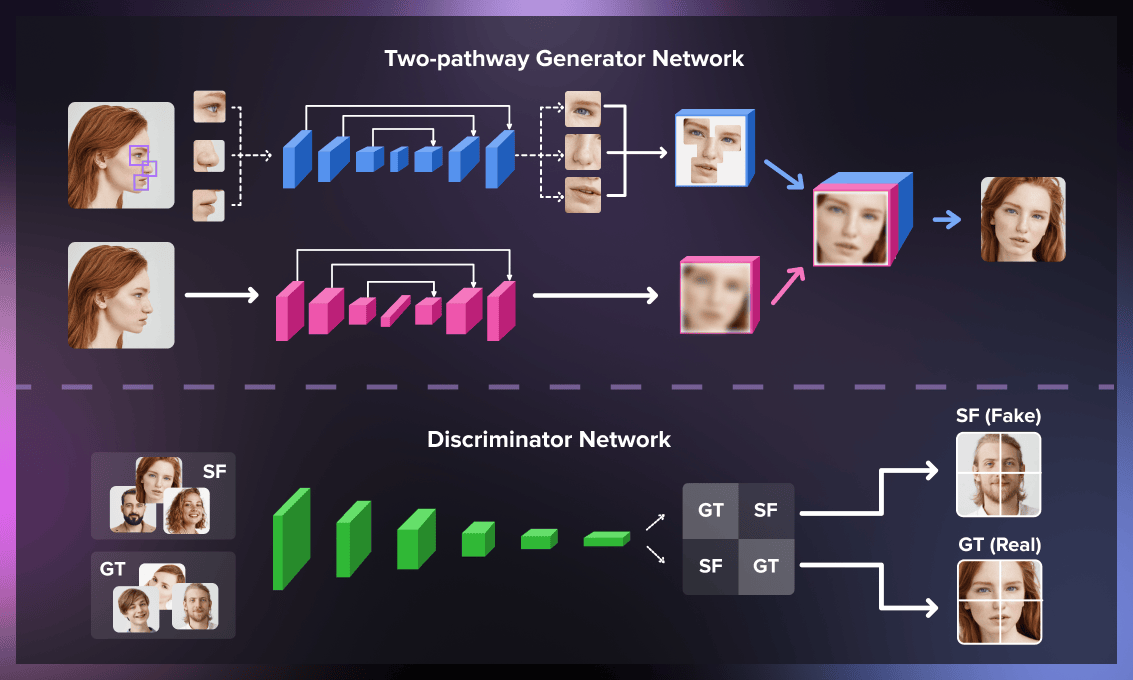
Once training is complete, the generator can create deepfakes by taking an input image or video and generating a fake version. In contrast, the discriminator can be used to detect deepfakes by evaluating the authenticity of an image or video. However, as the generator becomes more sophisticated, it becomes increasingly difficult for the discriminator to distinguish between real and fake pictures.
Watch the following video for a more detailed explanation:
There are two ways to create deepfake videos. The first involves manipulating the actions and words of the target by using an original video source. The second method is a face swap, transposing the person’s face onto someone else’s video.
Deepfake types
There exist several approaches to generating deepfakes, depending on the source of the manipulated material and the purpose.
-
Video deepfakes: This method uses a deepfake autoencoder based on neural networks to analyze the source video’s relevant attributes, such as facial expressions and body language. The autoencoder includes an encoder to encode these attributes and a decoder to impose them onto the target video.
-
Audio deepfakes: To create audio deepfakes, a GAN clones the person’s voice, creating a model based on vocal patterns. This model can then make the voice say anything desired. This technique is commonly employed in video game development.
-
Lip syncing: This technique involves mapping a voice recording to the video, making it appear that the person is speaking the words in the recording. This method is supported by recurrent neural networks and can add another layer of deception if the audio is also a deepfake.
Are deepfakes illegal?
As the potential harm from deepfakes becomes a growing concern for all continents, leading countries are establishing and updating regulations to safeguard people from the threat of misinformation. In the US, 2020 legislation required the National Science Foundation to research on the consequences of deepfakes and similar content and to develop methods to combat current and future technological equivalents. Additionally, DARPA, a defense agency under the Pentagon’s jurisdiction, is working with prominent research institutions nationwide to proactively address the issue of deepfake threats.
In March 2023, China introduced new regulations on deepfakes, which are regarded as the most comprehensive in the world. These regulations prohibit the creation of deepfakes without user consent and require the inclusion of clear identification that the content has been generated using AI. The European Union plans to revise its entire AI policy in 2023, which will include measures for detecting deepfakes.
While authorities work to implement directives and regulatory measures, there are simple methods we can use to recognize deepfakes.
How to identify a deepfake?
To determine if content has been manipulated, it’s essential to pay close attention to details.
Check the source
Deepfakes are often created using existing images or videos, so comparing the source content with the deepfake can reveal inconsistencies, such as differences in lighting, background, or perspective.
Look for visual artifacts
Deepfakes can often have subtle visual artifacts, such as blurry or distorted edges around the face or body, inconsistent lighting or shadows, or irregularities in eye movement.
For example, in an image that depicts French President Emmanuel Macron working as a garbage collector during France’s pension reform strikes, the illegible writing on the trash bags can be a clue that the image is fake.
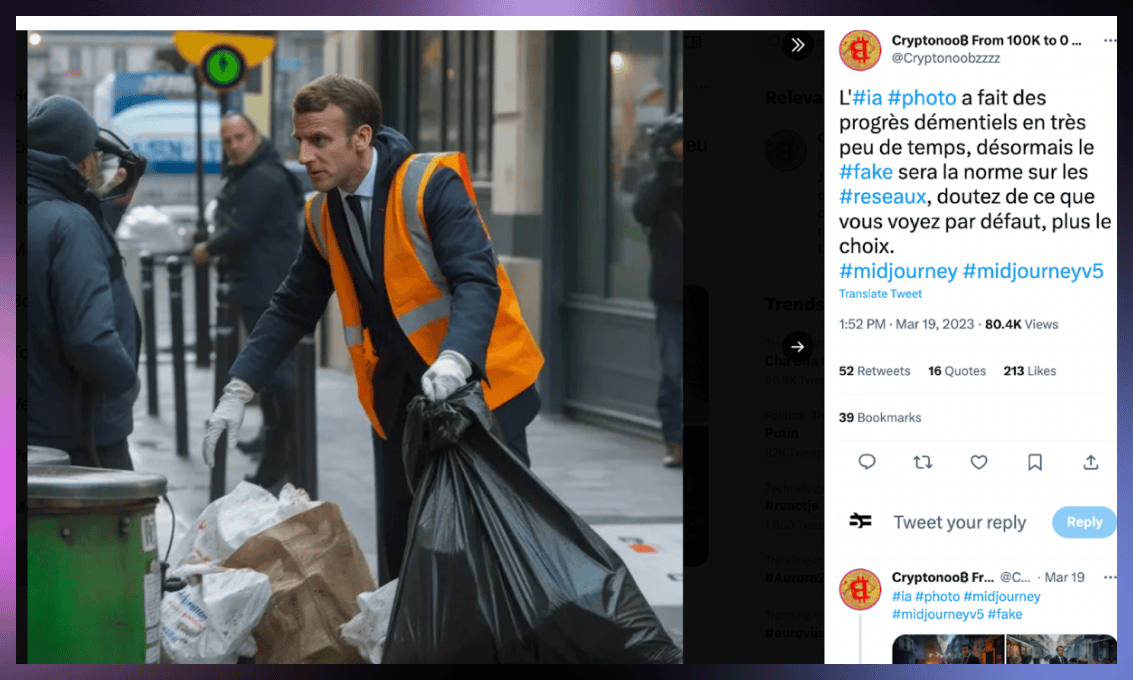
Listen to the audio
Audio deepfakes can be detected by listening for inconsistencies in tone, pitch, and background noise, which can indicate that the audio has been manipulated.
Examine facial and body movements
Deepfakes may not always mimic natural facial or body movements, such as blinking or breathing, as accurately as real videos do.
Deepfake videos typically share two distinct features: abnormal eye movements and audio that does not match the person’s mouth movements. In a 2019 video where Donald Trump denounces his impeachment proceedings and abruptly asserts that Jeffrey Epstein did not commit suicide, an apparent discrepancy between his spoken words and lip movements can be observed.
Advanced AI solutions for deepfake recognition
With the proliferation of fake news, the need for deepfake recognition technology has become increasingly important, especially in cases where people struggle to differentiate between real and fabricated information. Here are some platforms we’ve compiled to help you identify manipulated content.
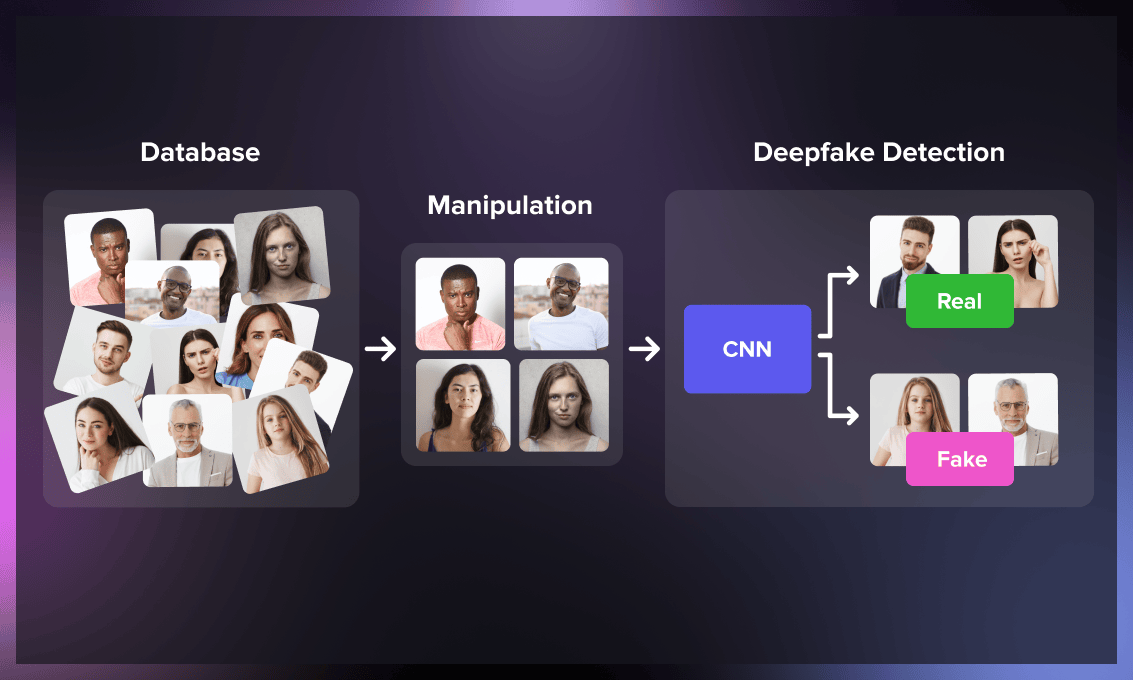
- Sensity is a specialized online platform for detecting deepfakes. You can upload files in various formats, including MP4, JPEG, and TIFF, which then undergo a scanning process. Sensity claims it can identify forgeries within a second with 98.1% accuracy, specifically targeting fraud techniques like face-swapping.
- Microsoft Video Authenticator Tool, released in the run-up to the 2020 US election, analyzes a still photo or video to determine the likelihood that it has been manipulated, providing a confidence score to that effect. Specifically, it can identify the blending boundary of deepfakes and other minor elements that may be undetectable to the human eye. The tool was created using the public dataset from Face Forensics++ and has been extensively tested using a deepfake detection challenge dataset.
- Deepware Scanner is an open-source forensic tool specifically designed to detect deepfakes. What sets this scanner apart is that it has been tested on various data sources, including organic and live videos. The scanner is powered by EfficientNet-B7, a convolutional neural network architecture model that uniformly scales all CNN dimensions, increasing accuracy, and overall cost-efficiency. The developers of Deepware Scanner emphasize the importance of community support in combating deepfakes. That is why they keep the project open-source and encourage researchers to contribute.
- Deepfake-o-meter is an online platform designed for deepfake detection, enabling users to perform various tasks, such as analyzing suspicious video files, running individual algorithms on different servers, and comparing the effectiveness of different algorithms on a single input. Users can upload a video via a URL link or as a file, with a maximum size limit of 50 MB. The platform utilizes Xception, ClassNSeg, EfficientNet-B3, CNNDetection, spatial pyramid pooling, and mesoscopic image properties analysis.
Below is a leaderboard of ML models collections for deepfake detection, with links to the code.
Model | Recommended dataset | Link to code |
Cross Efficient Vision Transformer model | DFDC | Link |
Face Forgery Generation and Detection | FaceForensics++ | Link |
XceptionNet | FaceForensics | Link |
The future of deepfakes
As deepfake tools advance, it is important to continue researching and developing effective methods to detect and combat deepfakes. While this technology has the potential for a positive impact, it holds a lot of risks and implications for society’s security, privacy, and creative potential. We must ensure it is managed responsibly. Ultimately, the future impact of deepfake technology will depend on the choices we make today and our ability to balance its benefits and risks.
Further reading: