We have already entered the era of AI. The next step would be Artificial General Intelligence (AGI), which does not yet exist and remains a topic of debate within the scientific community. In this article, we will explore the concept of AGI and examine the current technological capabilities for its development.
What types of AI exist?
To better understand the concept of Artificial General Intelligence, let’s first examine the forms of AI that we are already using today. These types of AI, designed to achieve specific goals, fall into the category of narrow AI.
Narrow AI
Narrow AI, also known as weak AI and Artificial Narrow Intelligence (ANI), is the only type of artificial intelligence that has been successfully developed to date. It refers to AI systems designed to perform a specific task or a set of closely related tasks. ANI does not replicate human intelligence but rather simulates human behavior within a limited range of parameters and contexts. Examples include image generation and recognition, natural language processing, computer vision, etc. AI systems in self-driving cars, recommendation engines, Siri, Google Assistant, and Alexa are all forms of narrow AI.
Over the past decade, narrow AI has achieved significant breakthroughs, largely due to advancements in machine learning and deep learning. For instance, AI systems are now used in medicine to diagnose cancer and other diseases with high accuracy.
Narrow AI is powered by natural language processing (NLP), which empowers it to comprehend and process human language.
ANI can be divided into two types: reactive AI and limited memory AI.
- Reactive AI cannot store data or memories and responds to stimuli solely based on immediate inputs, such as the movement of a chess piece on a virtual chessboard. Other examples include spam filters and recommender systems that suggest what to watch next.
- Limited memory AI can store knowledge, learn from it, and perform new tasks based on that learning. This type of AI is used for making predictions and forecast trends in various fields, from finance to weather and manufacturing safety. It is the most common type of AI today, found in applications such as chatbots and self-driving cars.
What is AGI?
Artificial General Intelligence (AGI) is AI with capabilities equivalent to or surpassing those of a human. It encompasses the ability to learn, understand, and apply knowledge across diverse domains.
AGI is also known as strong AI, full AI, human-level AI, or general intelligent action. However, some academics use the term “strong AI” specifically for computer programs that have sentience or consciousness.
Although still theoretical, AGI might one day replicate human-like cognitive abilities such as reasoning, problem-solving, perception, learning, and language comprehension. However, we are not there yet.
What are AGI benchmarks?
There are multiple benchmarks for testing the abilities of AI, the achievement of which would indicate that artificial intelligence has reached the level of AGI. Below are some examples. No AI tool has passed any of these tests so far.
Turing Test
The Turing Test assesses whether a machine is able to demonstrate human-like intelligence by evaluating whether it can engage in conversation indistinguishably from a human. If an evaluator cannot reliably tell the difference between the machine and a human, the machine is said to have passed the test.
Stanford Question Answering Dataset (SQuAD)
SQuAD evaluates a machine’s reading comprehension by testing its ability to answer questions based on a provided passage of text. The benchmark consists of questions formulated by crowd workers on a set of Wikipedia articles, with answers typically spanning text from the passages.
MS MARCO (Microsoft MAchine Reading COmprehension)
MS MARCO evaluates the capabilities of a machine in understanding and answering real-world questions by providing a dataset of questions along with passages retrieved from web documents. This benchmark is designed to test both the retrieval of relevant information and the ability to generate accurate and contextually appropriate answers.
ARC-AGI
Abstraction and Reasoning Corpus for Artificial General Intelligence (ARC-AGI) is the most comprehensive test to date. It was developed in 2019 by the creator of Keras, François Chollet.
ARC can be seen as a general artificial intelligence benchmark, as a program synthesis benchmark, or as a psychometric intelligence test. It is targeted at both humans and artificially intelligent systems that aim at emulating a human-like form of general fluid intelligence. —Source
The ARC-AGI test includes input-output tasks featuring puzzle-like grids, each square being one of ten colors. The grids can vary in size, ranging from 1x1 to 30x30.
To complete a task, the test-taker must generate a pixel-perfect output grid that matches the required final output, including selecting the correct dimensions for the output grid.
On average, a human completes 85% of tasks (where the output image for a new example matches the author’s), while LLMs only solve a few percent. The best systems, optimized for a similar class of tasks, achieve around 34%.
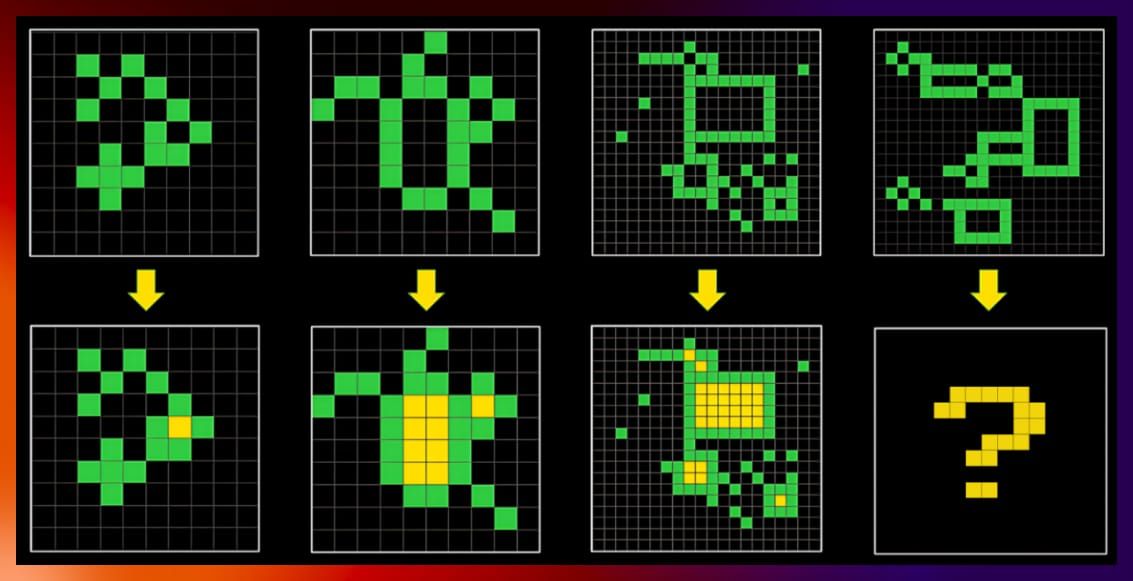
What’s the difference between AI and AGI?
There’s a fundamental difference between current AI and AGI. Models like ChatGPT and DALL-E, though remarkable, are essentially advanced prediction machines trained on vast amounts of data to generate accurate responses. They excel in specific tasks but lack human-level creativity, logical reasoning, and sensory perception. In contrast, AGI would exhibit cognitive and emotional abilities, such as empathy, and might even understand the meaning behind its actions.
In 2023, researchers at Google DeepMind proposed a framework to classify AGI into five levels:
- emerging
- competent
- expert
- virtuoso,
- superhuman
A competent AGI performs better than 50% of skilled adults in various non-physical tasks, while a superhuman AGI surpasses 100%. They consider large language models like ChatGPT or LLaMA 2 examples of emerging AGI.
Although Artificial General Intelligence is not yet available, there are narrow AI systems that approximate or even surpass human abilities in specific areas. Current AI research focuses on these systems to explore the potential of future AGI. Here are some examples:
- IBM’s Watson: Capable of performing complex calculations beyond the reach of average computers, Watson combines immense computing power with AI to tackle tasks like modeling the Big Bang theory or the human brain.
- ROSS Intelligence: Dubbed the “AI attorney,” ROSS can analyze data from about a billion text documents and provide precise responses to complex legal questions in under three seconds.
- AlphaGo: This AI excels at playing the board game Go, having defeated world champion Lee Sedol in a five-game match in 2016.
- Generative Pre-trained Transformers (GPT-3 and GPT-4): Language models from OpenAI that generate human-like text, emulating general human intelligence, though sometimes with flaws.
- Music AIs: For instance, Dadabots generates streams similar to a given body of existing music.
When will Artificial General Intelligence be achieved?
Despite varying opinions, many academics and researchers believe there is a possibility of achieving human-level artificial intelligence within the next decade. Richard Sutton, a professor of computer science at the University of Alberta, said in a talk:
Understanding human-level AI will be a profound scientific achievement (and economic boon) and may well happen by 2030 (25% chance), by 2040 (50% chance)—or never (10% chance).
Some believe AGI is still decades away. For instance, MIT roboticist and iRobot cofounder Rodney Brooks estimates AGI won’t be achieved until 2300.
Although the timeline for AGI’s emergence is uncertain, its arrival will significantly impact our lives, businesses, and society.
What are the requirements for AGI?
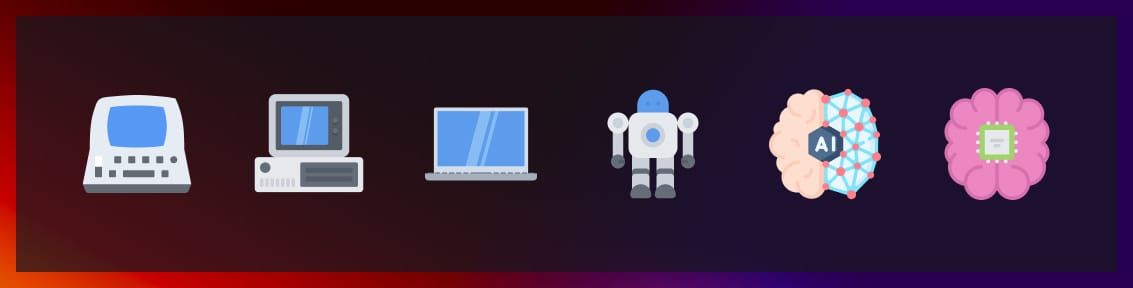
To reach the level of AGI, AI must possess the following abilities:
Abstract thinking: Reasoning about ideas, principles, and relationships that are not tied to specific instances or physical objects. Abstract thinking makes AGI capable of solving problems that are not explicitly defined. This ability is crucial for tasks such as strategic planning, philosophical reasoning, and scientific discovery.
Example: Developing a new scientific theory
- AGI analyzes existing scientific data and literature, identifies patterns and inconsistencies, and formulates a new hypothesis or theory that explains the observed phenomena. This requires reasoning about abstract concepts such as energy, force, and time, and proposing relationships and principles that are not directly observable.
Background knowledge: This foundational knowledge, including facts, concepts, and understanding of various domains, accumulated over time, is essential for interpreting new data, filling in information gaps, and providing relevant and accurate responses.
Example: Diagnosing a medical condition
- AGI uses its extensive medical knowledge base, including anatomy, symptoms, diseases, and patterns to diagnose a patient’s condition. It interprets the patient’s symptoms, medical history and test results, summarizes them, and provides a relevant and accurate diagnosis along with potential treatment options.
Common sense: The basic level of practical knowledge and reasoning concerning everyday situations that most humans possess intuitively. Common sense helps AGI navigate routine tasks and interactions by understanding what is typical or expected in various contexts. This includes recognizing implications and making reasonable assumptions.
Example: Planning a daily schedule for a household
- AGI considers the daily routines, preferences, and needs of each household member to create a practical and efficient schedule. It includes common-sense tasks such as preparing meals at appropriate times, ensuring time for work, school, and leisure, and accounting for typical human needs like sleep and relaxation.
Understanding cause and effect: The ability to recognize and predict how actions and events influence one another, identifying causal relationships. Understanding cause and effect allows AGI to anticipate the consequences of actions, reason about the dynamics of systems, and make informed decisions based on potential outcomes.
Example: Managing traffic flow in a city
- AGI analyzes traffic patterns, road conditions, and events like accidents or construction. It predicts the impact of these factors on traffic flow and makes real-time adjustments to traffic signals and rerouting recommendations to minimize congestion and improve travel times.
Transfer learning: The ability to apply knowledge and skills learned in one context to new, different contexts. This involves generalizing learning from specific tasks to broader applications. It allows AI to leverage previous experiences to tackle new problems and makes it capable of continuous improvement across various domains.
Example: Adapting a customer service chatbot to a new industry
- AGI applies knowledge and conversational skills learned from handling customer service in the retail industry to a new domain, such as banking. It generalizes from previous interactions to understand new types of customer inquiries, regulatory requirements, and service protocols, providing accurate and helpful responses without extensive retraining.
Higher-level capabilities anticipated for AGI
After achieving the capabilities described in the previous section, AGI is expected to advance to the next stage, where it will be able to perform even more complex tasks. They include:
Handling various types of learning and algorithms
AGI must be proficient in employing and integrating multiple learning paradigms and algorithms, such as supervised learning, unsupervised learning, reinforcement learning, and deep learning.
Creating fixed structures for all tasks
AGI needs to develop and utilize stable frameworks or schemas that can be applied across various tasks. These structures might include ontologies, hierarchical models, or standardized processes that guide AGI’s approach to task execution.
Understanding symbol systems
AGI must be able to interpret and manipulate symbolic representations, such as language, mathematics, logic, and diagrams to comprehend instructions, perform calculations, and engage in abstract thinking.
Using different kinds of knowledge
AGI must integrate and apply various types of knowledge, including procedural (how to do things), declarative (facts and information), and conditional (understanding when and why to apply certain knowledge).
Understanding belief systems
AGI must recognize and interpret belief systems, including the values, assumptions, and worldviews held by individuals and groups. This understanding is essential for effective communication, social interaction, and ethical decision-making.
Engaging in metacognition and utilize metacognitive knowledge
AGI must have the ability to reflect on its own thinking processes (metacognition) and use this awareness to regulate and improve its performance.
Current challenges in AGI research
As we have mentioned, AGI is still in the theoretical stage due to multiple challenges that current technology does not yet allow us to overcome. These include making connections, possessing emotional intelligence, developing sensory perception abilities, and embedding ethical frameworks.
Making connections
Current AI models are confined to their specific domains and struggle to make connections across different fields. For instance, educational theories can be applied in game design to create engaging learning experiences, and theoretical education can be adapted to real-life situations. Deep learning models, however, require extensive training with specific datasets to function reliably with unfamiliar data, limiting their adaptability.
Emotional intelligence
While deep learning models show potential for AGI, they have not yet demonstrated genuine human creativity, which involves emotional thinking. Neural networks currently cannot replicate this aspect. For example, humans respond to conversations based on emotional cues, whereas NLP models generate responses based solely on linguistic patterns and datasets they are trained on.
Sensory perception
AGI development necessitates AI systems’ ability to physically interact with and perceive the external environment similarly to humans. This includes distinguishing shapes, colors, tastes, smells, and sounds accurately. Existing computer technologies require significant advancements to achieve this level of sensory perception and interaction.
Ethical considerations
As AGI systems may be required to make decisions with moral and ethical implications, it is important to embed ethical frameworks within their decision-making processes to ensure they act in ways that are ethically justifiable.
Advances that could accelerate AGI development
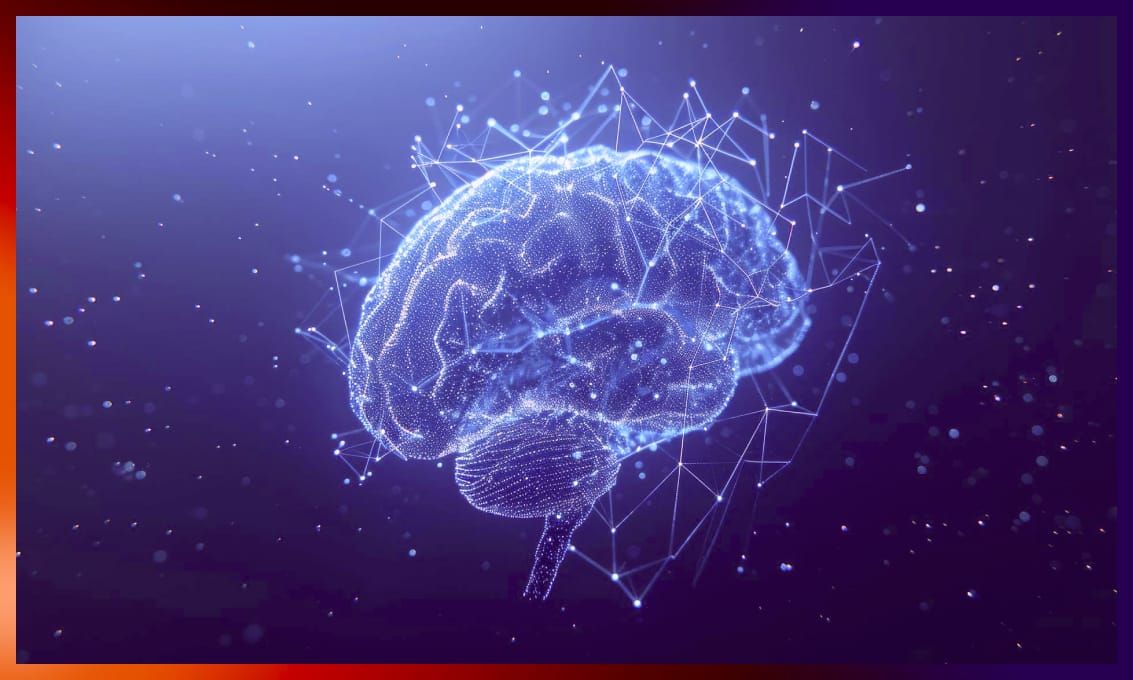
Recent progress in AI has been driven by advances in algorithms, computing, and data. Future developments in these areas could speed up the achievement of AGI:
Algorithmic advances and robotics
Embodied cognition: Experiencing the physical world is crucial for developing human-like cognition. That is why researchers are exploring embodied cognition, where robots learn quickly from their environments through multiple senses, similar to how humans learn as children.
Generative AI technologies: Latest AI-based robot systems use large language models (LLMs) for advanced natural language processing and large behavior models (LBMs) to emulate human actions. These models train on large datasets of human behavior, enabling robots to perform various tasks with minimal specific training.
Built-in knowledge: Developing AI systems with inherent knowledge, similar to instinctual abilities of a baby fawn, deer, or bird, could significantly advance AGI. This might require shifting the focus from deep learning-based systems to fundamental cognitive research.
Computing advancements
Graphics Processing Units (GPUs) have been pivotal in recent AI breakthroughs due to their ability to handle visual data and train complex neural networks efficiently. Future advancements in computing infrastructure, including quantum computing, are essential. Quantum computing, while not yet ready for everyday use, holds promise for achieving AGI.
What are the energy costs of AGI?
AI models containing billions of parameters require substantial amounts of energy for training. According to AI company Numenta, OpenAI’s previous GPT-3 system reportedly consumed 936 megawatt hours (MWh). For context, the US Energy Information Administration estimates that an average household uses about 10.5 MWh annually. Therefore, training GPT-3 consumed the same amount of energy as approximately 90 households use in a year. Larger models will necessitate even more energy.
Alex de Vries, a PhD candidate at VU Amsterdam, first calculated the energy expenditure of Bitcoin for his blog, Digiconomist. He used Nvidia GPUs — considered the gold standard in AI hardware — to estimate the sector’s global energy usage:
AI servers are power-hungry devices. A single NVIDIA DGX A100 server can consume as much electricity as a handful of US households combined. Because of this, the electricity consumption of hundreds of thousands of these devices will start to add up quickly. While the supply chain of AI-servers is facing some bottlenecks in the immediate future that will hold back AI-related electricity consumption, it may not take long before these bottlenecks are resolved. By 2027 worldwide AI-related electricity consumption could increase by 85.4–134.0 TWh of annual electricity consumption from newly manufactured servers. This figure is comparable to the annual electricity consumption of countries such as the Netherlands, Argentina and Sweden. —Source
Efforts are being made to improve AI energy efficiency through specialized hardware and techniques, such as DeepMind’s data center cooling system. These initiatives aim to lower the energy consumption of AI technologies. However, at this point, no efficient solution to this problem exists. Unless it is solved, humanity simply won’t have enough energy to train AI in a way that could evolve into AGI.
What would be the next stage after AGI?
After AGI is achieved, its natural self-development would result in the emergence of Artificial Superintelligence (ASI).
ASI is a hypothetical form of AI that doesn’t merely imitate or comprehend human intelligence and behavior but achieves self-awareness and surpasses human intelligence and capabilities.
The notion of superintelligence has been a central theme in dystopian science fiction, depicting scenarios where robots dominate, overthrow, or enslave humanity. In this concept, ASI evolves to such an extent that it not only understands human emotions and experiences but also develops its own emotions, needs, beliefs, and desires.
ASI would theoretically excel in all areas of human ability, including mathematics, science, sports, art, medicine, hobbies, and emotional relationships. With superior memory and faster data processing and analysis, ASI’s decision-making and problem-solving capabilities would far exceed those of humans.
Conclusion
AGI aims to reach human-level cognition, learning, and performing various tasks independently.
In 2024, the quest for artificial general intelligence became one of the most ambitious challenges in AI research. Although the timeline for achieving it is still uncertain due to current technological and energy limitations, overcoming them would result in exponential growth of AI capabilities and its potential evolution into AGI.
Whenever this happens, it will turn a completely new page in human history, and we need to prepare for it today.