Early detection of diseases associated with genetic disorders is one of the biggest concerns for modern medicine. Recent research states that people diagnosed with lung cancer at an early stage have a 57% chance to survive the next 5 years compared to the 3% survival rate of patients with diagnosed IV stage cancer. Early-stage detection of another scourge of humanity, Alzheimer’s disease, allows patients to change their lifestyles, participate in clinical trials, and treat the brain-degrading symptoms in advance, effectively prolonging their lives. While the genetic tests only help to evaluate the likelihood of late-onset Alzheimer’s, they are a good indicator for the appearance of early-onset Alzheimer’s as it’s caused by mutations in three distinct genes (recent study on DNA testing for early-onset Alzheimer’s disease).
There are a variety of disease detection techniques based solely on biological research and, although they can be very precise for investigating particular cases, usually they lack one very crucial thing – they require additional complicated medical tests performed on patients with a suspected disease. Such tests are too costly to be performed on a regular basis on healthy people. For instance, any person can take a regular blood test once in a while, but hardly anyone would want to undergo a whole-body MRI just as a precaution against cancer, not to mention invasive tests like biopsy.
Hence there is a great need to develop easy-to-perform tests which will accurately detect such diseases. For now, the most promising direction in this field is via genetics, although there are a number of tasks to solve before we arrive at the destination. And machine learning has proposed a lot of tools to help with those tasks, including API monitoring tools. In the article, I would like to cover some of the ML applications to genetics data, as well as the necessary information to understand them. It is worth noting that this field of data science is developing at an incredible pace, so treat the article as a starting point or a source of inspiration rather than a comprehensive review or a tutorial.
For a better understanding of the following material, I’d suggest you read this article by Rachel Lea Ballantyne Draelos which contains all the basics from a microbiology perspective presented in a simple and elegant way. Also, to keep the article rather concise, I’ll omit some of the details of how basic machine learning algorithms work and focus on particular models and applications.
Basics of genetics
Humanity’s journey into genetics started in 1866, when Gregor Mendel, also known as the “Father of Genetics”, proposed that characteristics are passed down from generation to generation. In the last 155 years, we’ve come a long way in understanding the nature of the genome to the point where we can even decipher and modify it. To cover all the details of the journey, several dozen volumes of an encyclopedia are required, but I’ll try to get by with just one paragraph.
The genetic information constitutes all the processes in any living organism and is stored in the organism’s DNA. DNA, in turn, is two “opposite” strings of 4 types of nucleotides (T, A, C, G) which are around 3 billion length. Almost all of the organism’s cells contain DNA. DNA is transcripted into RNA, which also contains 4 types of nucleotides (A, U, C, G). Each triplet of nucleotides of RNA, called a “codon”, encodes one of 20 amino acids with several exceptions used for “start” and “stop” of the encoding sequence. Proteins are constructed from amino acids and are the ultimate building blocks of all living creatures.
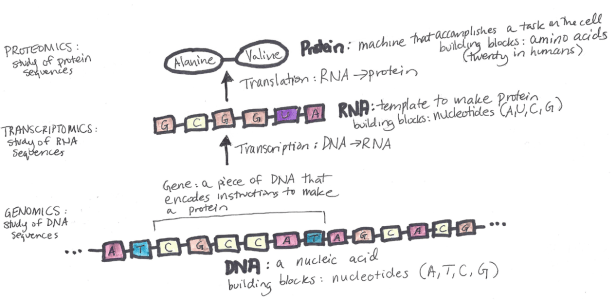
Note that the scheme above is very simplified, there are many different pitfalls since not all RNA sequences get translated into protein, and not all parts of RNA are used when constructing protein. In fact, only around 3% of all RNA sequences get translated into proteins, the other 97% are called non-coding RNA (ncRNA). ncRNA is sometimes referred to as the “dark matter” of the genome, as we know little about its properties. There are other multiple types of RNA, such as mRNA, tRNA, rRNA, and each of them has their own purpose in the process of constructing proteins. The whole flow of genetic information within a biological system is described in the Central Dogma of Molecular Biology, but modern research showed that even some of the dogma statements are not completely accurate.
Genetic mutations
Here’s the question for you: why are people so different from each other? Some are very tall, some can run tirelessly for miles, some have blue eyes or curly hair, and some are immune to HIV. Since the moment the first people appeared on the Earth, the variety of species traits keeps broadening with the growth of the population and passage of time. Why?
Genetics has an answer to the question. As we know, DNA is responsible for everything in our body. This leads us to an important insight – each person has a unique genome. Even further, cells within the same organism can have different DNAs (e.g. a phenomenon known as mitochondrial heteroplasmy). Although, of course, the difference in genomes of closely related species tends to be smaller than between dissimilar ones. Note that even the same DNA sequences can “be interpreted” differently. Such a process is described by the science field called epigenetics which I’ll cover in short later in the article.
The difference in genomes within the same species is caused by DNA mutations. In fact, we’re all born with DNA mutations, inherited from our parents (so-called “germline mutations”). However, you can obtain DNA mutations even during your lifetime. Usually, it happens because of errors during the DNA duplication process, but sometimes the mutations are caused by external factors such as UV radiation, chemicals, or viruses.
Most of the mutations are silent and do not explicitly affect you, some can even be beneficial, but there are few which can cause serious health problems. The latter, although quite rare, are the ones we hear about the most: genetic diseases, cancer, etc.
One of the basic mutations is called a single-nucleotide polymorphism or SNP, which is the simplest form of DNA variation among individuals. SNP is the substitution of one single nucleotide for another. It is estimated that SNPs occur at a frequency of 1 in 1,000 bp throughout the genome, where “bp” is a frequently used abbreviation for “base pair”, which is a pair of double-stranded nucleobases bonded by hydrogen connection. Although a single SNP rarely causes a disease, combinations of SNPs in particular genes may lead to various diseases, some of which you can check out in Table 1 of this paper.
The noble task of describing and cataloging different SNPs has been in the process of being resolved for many years. There are several publicly available SNP databases on the internet, such as OMIM, ClinVar, DisGeNET, and NCBI.
Detection of genetic disorders
One might ask: what’s so difficult in detecting gene-related diseases? Just take the whole genome of a person, look for the required pattern associated with the disease, make a conclusion. Done, here’s your prescription, take the medicine, and get well soon.
Well, as you might have guessed, it’s not that simple… First, let’s take into consideration the fact that the genome consists of roughly 3 billion nucleotides in a particular order. The process of obtaining the string of DNA is called DNA sequencing. It’s a very broad and challenging task which I won’t cover in too much detail as it’s out of the scope of the article. I’ll mention that currently the most frequently applied techniques are called “next-generation sequencing” or NGS. They read small portions of DNA strings and postprocessing algorithms assemble them together on the basis of their overlapping areas. A lot of effort has been put into making NGS as accurate and precise as possible, although there’s a number of issues occurring from the heterogeneity of the analyzed material. To tackle this problem, a “third generation” of DNA sequencing methods were invented. Such methods rely on single-molecule sequencing and are the most promising ones in the field at the moment. For more information on DNA sequencing, I suggest you check this paper.
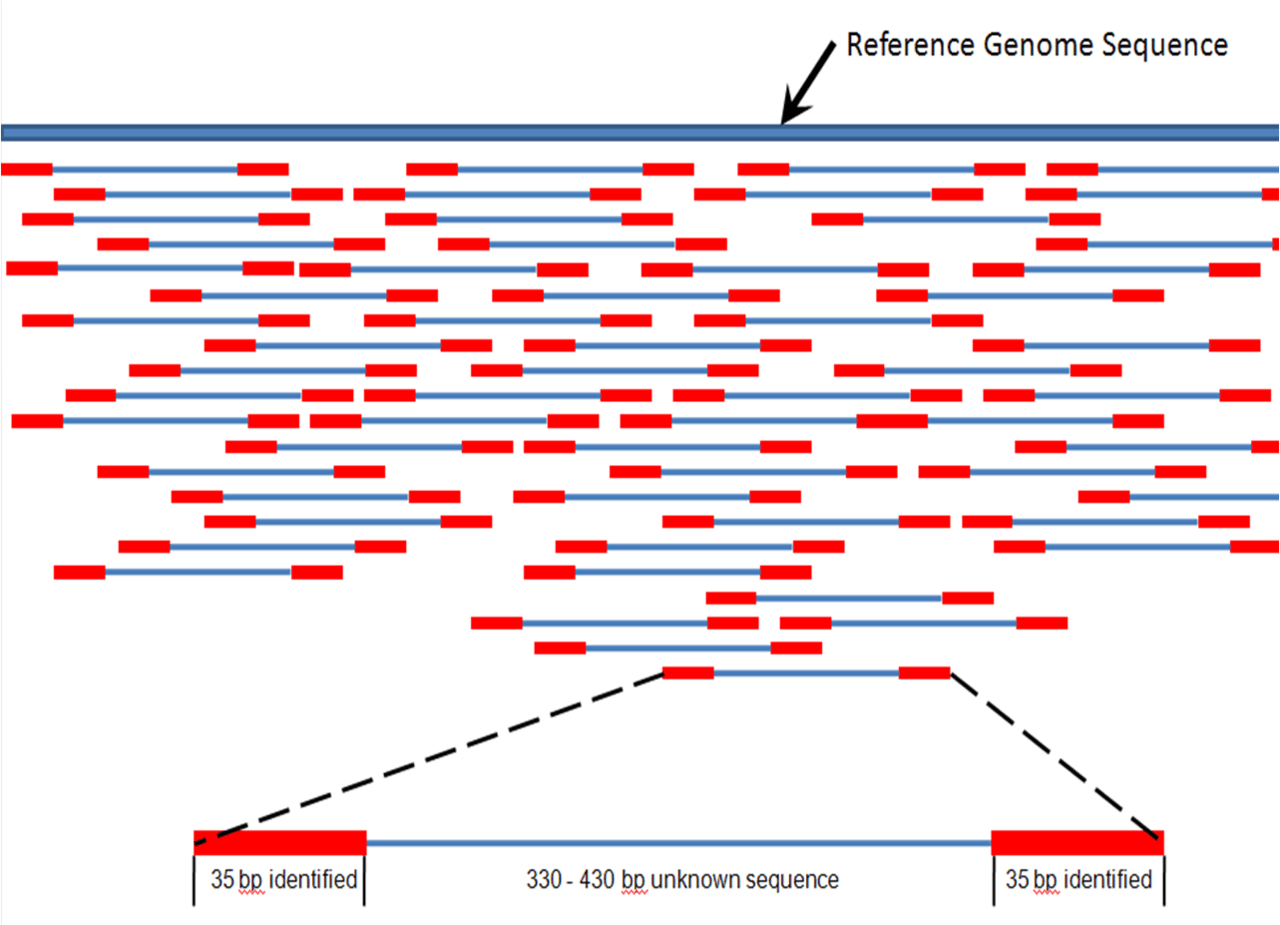
Even if you have obtained an accurate DNA string or multiple strings, the real challenge of detecting the disease has just started. As I mentioned above, gene mutations or combinations of mutations are responsible for multiple diseases. One of the problems is that some diseases (e.g. cancer) are caused by so many different and sometimes rare/unknown mutations that it’s almost impossible to manually look for all of them during the DNA testing. That’s the main reason why DNA testing for cancer is usually recommended only to people with a strong family history of certain types of cancer or people already diagnosed with cancer to check if it’s caused by inherited gene mutations in order to alert other family members. Another issue is that some of the genetic mutations leading to diseases occur in particular cells or tissues, so even one sample of genetic material is rarely enough to accurately detect the disease.
For those reasons, searching for mutated genetic material is not always the best approach to detecting the disease. For instance, there is a tumor protein p53 which is responsible for gene mutation suppression. Its associated gene TP53 is classified as a tumor suppressor gene. p53 functions are to repair damaged DNA, arrest cell growth, and initiate cell disintegration if everything went completely wrong. So, basically, p53 is responsible for stable and sustainable growth and renewal of the organism. However, mutated TP53 can stop producing p53 which often leads to terrible consequences such as cancer. The mutated TP53 is found in more than 50% of all human malignant tumors. Sadly, the mutated TP53 can mostly be found in the cancerous tissue and cannot be an efficient indicator of cancer.
Hence, the process of searching for diseases is narrowed down to searching for accurate disease indicators and correctly classifying them. Such indicators are called biomarkers and are measurable indicators of some biological state or condition. Medicine biomarkers are categorized into 3 types: molecular biomarkers (e.g. DNA, RNA, protein level), cellular biomarkers (e.g. histopathology), or imaging biomarkers (e.g. CT, MRI, X-ray, etc.). When diagnosing cancer, biomarkers can be a traceable presence of substances in the blood or tissue such as biomolecules produced either by the tumor cells or by other cells of the body in response to the tumor. Alzheimer’s most promising molecular biomarkers are amyloid-β (Aβ42), total tau (T-tau), and phosphorylated tau (P-tau) in cerebrospinal fluid. For rheumatoid arthritis, the rheumatoid factor (RF) and the anti-cyclic citrullinated peptide (anti-CCP) are considered to be good indicators. Note that biomarkers used in clinical practice have to meet certain strict criteria, e.g. specificity and sensitivity of at least 0.9, but criteria vary for different diseases.
AI in genetics
Luckily, the existing system of diagnosing genetic-related diseases is changing rapidly. The process of searching for disease biomarkers on different levels has become cheaper, faster, and more efficient due to advances in computational science, microbiology, and lab hardware. This, in turn, leads to larger databases of labeled medical images and studies. And, of course, such databases are perfect research sites for data scientists. Multiple tech universities, big companies, and AI startups are partnering with medical institutions in order to develop novel approaches to disease testing with the use of machine learning algorithms and, specifically, deep learning.
Deep learning is a family of high-level abstraction algorithms called artificial neural networks (ANNs) that are able to identify multiple structures and patterns in the data, frequently not detected by humans. Although the concept of deep neural networks occurred in the middle of the 20th century, only by the 21st century it gained general popularity due to advances in parallel computing and GPU acceleration. There are thousands of applications of deep learning to date, some of the most notable are in such fields as natural language processing and computer vision, where artificial neural networks have achieved a beyond human level of perception for multiple tasks. Here I’d like to show some of the recent examples of ANN application to genetics as well as potential further research.
Histopathology
Although conventional ML has been used in microbiology quite extensively for many years, deep learning has gained momentum rather recently. Convolutional neural networks (CNNs), one of the most developed areas of deep learning, have shown great results in the tasks of analyzing histopathological images of tissues.
The National Cancer Institute defines histopathology as “the study of diseased cells and tissues using a microscope.” Basically, a histopathological slide is a high-resolution microscope image of tissue from a disease-affected organ. CNNs have been used to classify images in general (e.g. presence of cancer), detect affected cells or areas, classify multiple cancer types, and even measure gene expression. In some cases, the quality of prediction is similar to or even exceeds that of pathologists, but there are several drawbacks to such detection methods. The main one is that in order to get the material for examination, the patient should undergo a painful surgical procedure – biopsy – hence it cannot be used for healthy people. Also, training CNNs for object detection and classification requires a lot of labeled training samples. In the case of histopathology analysis, images should be labeled by experienced pathologists and the diagnosis should be historically proven. There are a number of open-source datasets one can download from the internet, but the amount of samples is usually not that large, and there are not enough cases of rare cancer types.
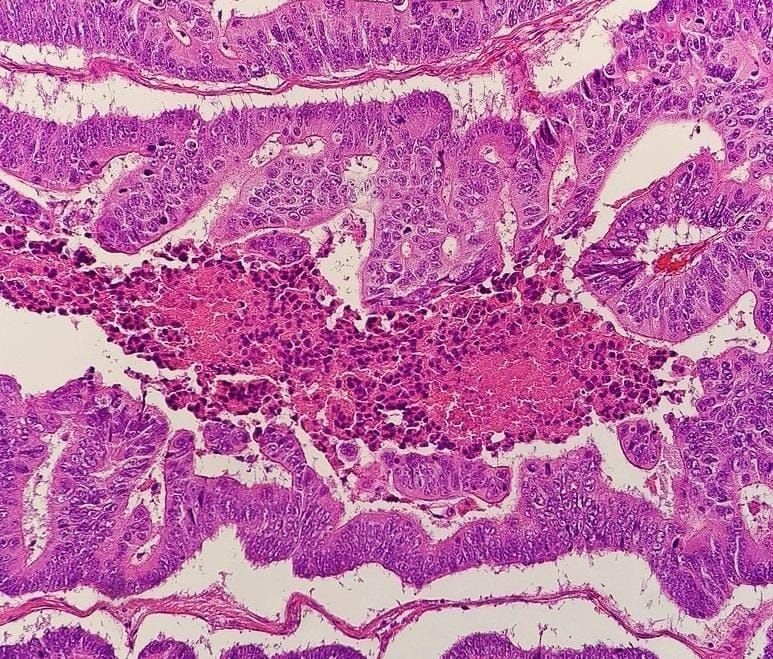
DNA variants
Understanding of genetic variants and mutations may be the key to developing better medical tests, and AI has significantly contributed to the field. One of the notable examples is DeepVariant by Google, an open-source deep learning-based variant caller. CNNs in DeepVariant take sequencing reads as input and are able to accurately reconstruct the genome while calling all the variants and sequencing errors. With DeepVariant’s predecessor model, Google Brain Team won the SNP performance precisionFDA Truth Challenge in 2016 and later developed a DeepTrio, an extension to DeepVariant that better tackles the inheritance properties of child/parent genomes.
ncRNA
Current evidence suggests that non-coding RNA plays a big role in tumorigenesis, Alzheimer’s disease, and cardiovascular diseases and can potentially be a great biomarker or a treatment target. Specifically, ncRNAs can affect cancer cell fate and survival through a variety of different mechanisms, including transcriptional and post-transcriptional modification, chromatin remodeling, and signal transduction. In short, ncRNAs create a complex network of mutual interactions and act as oncogenes or tumor suppressors. Unveiling the properties of ncRNA and its subtypes is among the hottest topics in genomics and especially its connection to diseases, but even distinguishing ncRNA from coding RNA parts and from one another is a really hard task. ncRNAs vary in forms, lengths, and properties, and there are a number of great projects tailored for ncRNA classification with the use of deep learning, but they cover a smaller part of the whole challenge. A lot of future work is needed to fully understand ncRNA and make use of it in clinical practice, but we’re on the right track! For more details on the ncRNA and its classification with ML, I’d suggest you read this great paper that covers the most successful approaches in the previous few years.
Circulating DNA and RNA
To develop highly accurate blood tests (also known as “liquid biopsy”), one will require an accurate blood-borne disease biomarker. Among the most promising ones are circulating free DNA (cfDNA) and circulating RNA (cfRNA). cfDNA and circulating RNA are extracellular fragments of DNA and RNA respectively, which can be found in blood, urine, synovial fluid or saliva. Some healthy cells also release genetic material, but elevated levels of cfDNA/cfRNA are usually an indicator of different ailments. Although the functions of cfRNA are less explored, there’s some great ongoing biological research in the field of Alzheimer’s, cancer, and Parkinson’s detection using circulating non-coding RNAs as biomarkers. I wanted to mention this field as it offers great opportunities for AI applications, although there are very few present to date (here’s one example of testing multiple machine learning algorithms for detecting Alzheimer’s, based on circulating miRNA). The type of cfDNA relevant to diagnosing cancer is circulating tumor DNA (ctDNA). ctDNA originates directly from the tumor or from circulating tumor cells, hence it can be useful not only for detecting cancer but also for analyzing the entire tumor genome as ctDNA carries its parts. The biggest challenge to date is that the concentration of target DNA in plasma can be quite low, so proper detection and sequencing methods are required to accurately evaluate the presence of ctDNA. ANNs are successfully used to help detecting ctDNA, for instance, this paper proposes a highly accurate CNN which detects the mutations of cfDNA relevant to lung cancer based on the collected genetic material from a liquid biopsy.
There are more uses of cfDNA for different purposes. If you are interested in the known properties of cfDNA, I’d suggest you check out this source for more insights. Also, here is a list of available cfDNA datasets for your experiments.
.png)
DNA methylation
Epigenetics is a second layer of information encoded onto the genome that guides genomic function and activity. Epigenetics acts through two mechanisms: modifications to chromosomal proteins that alter the 3D conformation of the genome and/or protein-DNA interactions and chemical modification of the DNA strand itself. The best characterized chemical modification of DNA is the methylation of cytosine to 5-methylcytosine (5mC) that occurs almost exclusively in the context of a cytosine base linked by the DNA phosphate-backbone to guanosine, termed a CpG site. Focal and global hypermethylation are tightly connected to processes in cancerous tissues and can be observed even at the early stages of the disease, hence they are a very useful input to ML models for disease prediction. Calculating the DNA methylation is usually closely related to ctDNA testing. While the task of assessing “average” methylation of the collected sample is solved quite well, such an approach is not always useful in practice due to the heterogeneity of collected material and the high background of non-target DNA. There’s a number of FDA-approved tests measuring methylation for clinical practice, most of which are listed in this great overview of modern approaches to measuring methylation of DNA, but the task of measuring methylation on a single-cell level still presents some difficulties. For instance, modern methods for single-cell methylation analysis such as scBS-seq are limited by moderate CpG coverage at around 20%. One of the approaches to resolve this issue is proposed in this paper where authors developed a combination of CNN and RNN based on bidirectional GRUs called DeepCpG, which is able to predict methylation states of missing CpG sites from DNA reads. DeepCpG was considered a state-of-the-art model for a long time, although more recent deep learning approaches suggest better results, such as the MRCNN convolutional network.
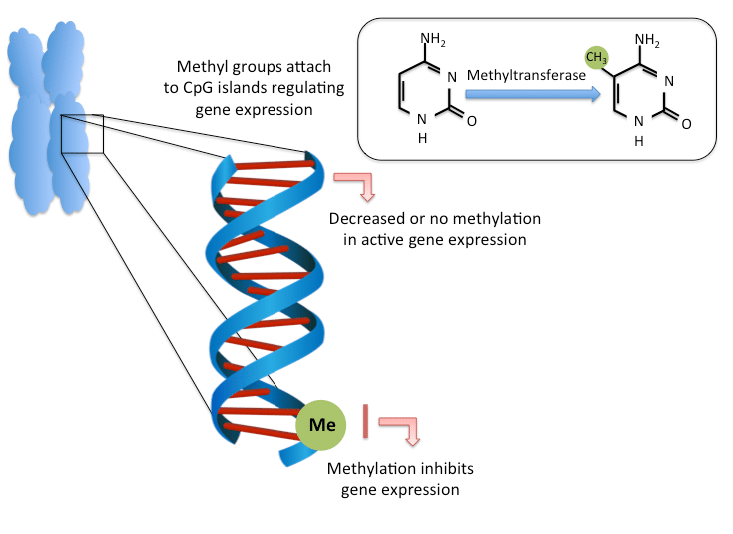
Miscellaneous
Artificial neural networks have also been successfully used to detect and identify virus DNA, design drugs and antibodies. The topic is closely related to the current article, but since it’s rather wide, I intend to cover it separately in future posts.
Note that there are multiple applications of ML for disease detection applied to different medical tests. Computer vision CNN techniques for CT and MRI medical images and biopsies, signal processing RNNs for electrocardiography and electroencephalography, and many others.
Conclusion
AI in medicine is constantly evolving and changing. I tried to cover a small part of it and provide you with a nice starting point. Now, I hope you have an impression of how modern AI techniques can be applied to the field of genetics and detecting genetic diseases. Do not assume that I’ve listed all research areas and take in mind that most of the aforementioned applications and models can be improved in many ways. I pursued a goal to inspire you to dive into the field, push the boundaries, and ultimately make our world a better place! There are already some great advances, but even more questions are yet to be answered.