The global big data and business analytics market size reached $198.08 billion in 2020 and is expected to triple by 2030. This fast growth shows that it’s crucial for businesses to adopt data analytics strategies to gain advantage in competitive markets.
In this article, we take a look at the data analytics trends in 2024 that you need to incorporate into your business strategy to maintain an edge.
What is data analytics?
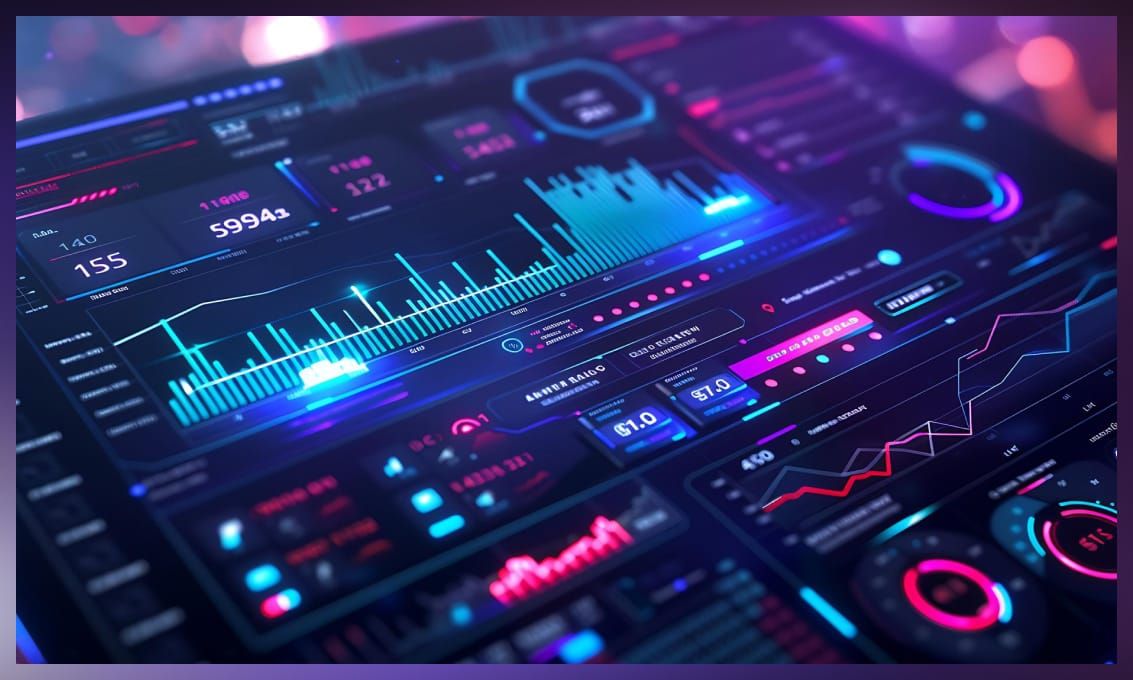
Data analytics refers to the process of examining raw data with the purpose of drawing conclusions about that information. It involves various techniques and tools to transform, organize, and model data to discover useful information, support decision-making, and provide actionable insights.
There are various types of data analytics:
- Descriptive analytics. It focuses on summarizing historical data to understand what happened in the past.
- Diagnostic analytics. Looks at past data to determine why something occurred.
- Predictive analytics. Uses historical data and statistical models to predict future outcomes.
- Prescriptive analytics. Recommends actions you can take to affect desired outcomes.
Data analytics is widely used across different industries including business, healthcare, marketing, and finance to improve operational efficiency and guide strategic decisions.
How has the data analytics landscape changed in recent years?
Lately, the market of data analytics has changed significantly. These are some of the contributing factors:
LLMs emergence
In recent years, the data analytics market has undergone significant changes, due to the emergence and strengthening of large language models (LLMs). Previously, data analytics relied on concrete and formal sources such as financial reports and stock exchange data. Today, technology enables effective analysis of more abstract and diverse sources, including meeting transcripts, news, and various public documents. These data have become more accessible due to transparency and anti-corruption laws, which require open publication of information.
Increased data availability
The rise of social media and mobile technology has resulted in vast amounts of user-generated data. In fact, the total amount of data created, captured, copied, and consumed globally is forecasted to grow to more than 180 zettabytes. The growth was accelerated by the pandemic, with more people working and learning from home.
However, alongside this, a new problem emerged: potential inaccuracies and unreliability of information. AI is actively used in various sources, which can lead to data theft and errors. While analysts mainly relied on official data in the past, they now need to learn to work with potentially unreliable information.
Additional regulations
An additional factor is the new regulations regarding personal data. For example, the European Union has introduced laws concerning cookies and restrictions on what data can be used for model training. When analyzing data such as tweets, it is important to consider in which jurisdiction they were collected and how lawful their use is.
In the future, it is likely that regulations that concern private data and data used to train generational artificial intelligence will become even more strict, especially in Europe, where there is already an existent trend.
Cultural shift
Many organizations now embrace a data-driven culture, where data is central to decision-making processes. This cultural shift has been driven by the recognition of data as a critical asset. Data preservation and validation have become crucial aspects. Companies now pay more attention to the quality of their datasets, striving to avoid generated data. For improving analytics quality, it is preferable to have fewer but more reliable records. This requires a meticulous approach to data collection and improving metrics by enhancing data quality.
Moreover, over the past three years, companies have realized the importance of data, leading to an increase in its value and complexity of access. Data that was previously considered publicly available now often requires purchasing rights for its use. This is especially noticeable on platforms like Twitter, where collecting data without permission can lead to bans and legal consequences.
The market also sees a growing demand for unique datasets, especially for rare languages or niche industries. Previously, there were companies providing such data in large quantities, but today, while data is available, the opportunities for analysis are becoming fewer. Companies prefer to avoid sharing data indiscriminately and focus on analyzing it more selectively.
Finally, a significant trend has been the focus on diversity and inclusivity in data. Companies aim to collect data that reflects diversity and ensures inclusivity, which is important for creating more accurate and representative models.
Top data analytics trends
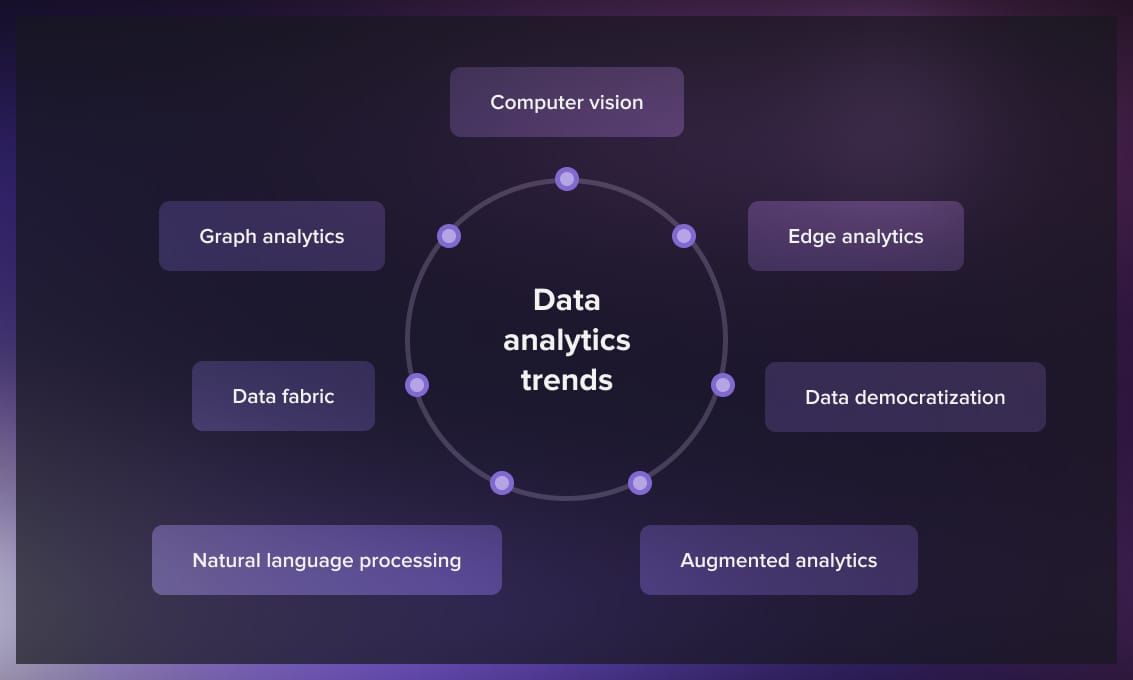
Organizations that leverage these trends are better positioned to gain competitive advantages and make informed decisions.
1. Edge analytics
The ARM architecture has a long history, with principles dating back 100 years and initially used in bionics. Today, this technology is advancing rapidly in computing devices. The popularity of ARM-based devices like smartwatches and smartphones is growing, and the possibility of ARM-based servers and computers is on the horizon. This evolution means that software developers may no longer need to rewrite and recompile software for different processor architectures, significantly reducing the complexity and workload.
With the widespread adoption of ARM architecture, coding becomes simpler and more efficient. A game or application developed for one ARM-based device can run seamlessly across various devices, from mobile phones to servers, ensuring a consistent and efficient user experience. The reduction in energy consumption and increased efficiency of ARM chips also contribute to the sustainability of computing technologies.
In addition to hardware advancements, the development of compact large language models (LLMs) has further enhanced edge analytics. Models like LLaMA can run on mobile phones, allowing for sophisticated data processing directly on the device. This capability reduces the need to collect and process vast amounts of data on centralized servers, shifting the focus to data processing at the edge.
Gartner predicts that more than 50% of critical data will be created and processed outside of the enterprise’s data center and cloud by 2025.
2. Data democratization
By leveraging techniques such as Retrieval-Augmented Generation (RAG) and knowledge maps, companies can create intelligent systems that provide employees with precise answers and easy access to necessary documents and data.
Training chatbots on internal data involves creating a system that understands the structure and location of documents within the company. This process includes aggregating various types of documents and data sources across the organization, as well as allowing the chatbot to fetch relevant documents and information from a vast database in response to specific queries.
Developing a knowledge map helps in structuring and indexing the data, making it easier for the chatbot to locate and provide the right information. Knowledge maps visually represent the relationships between different pieces of information, guiding the chatbot in understanding the context and relevance of the data.
One of the major challenges in deploying such chatbots is ensuring proper access control to sensitive information. For example, by implementing RBAC (role-based access control) that ensures employees only have access to data relevant to their roles. The chatbot needs to be integrated with the company’s identity and access management (IAM) system to enforce these controls.
A Harvard Business Review survey found that 97% of business leaders report that democratizing data is crucial to the success of their business. But only 60% of them say their organizations are effective at granting employees access to data and the tools they need to analyze it.
3. Augmented analytics
LLMs have revolutionized how we process and interpret vast amounts of information. With their advanced natural language understanding, these models can effectively analyze news articles, identifying key trends, sentiments, and insights that are valuable for businesses and researchers.
LLMs serve as expert tools in extracting and annotating visual data. This process, which traditionally required human intervention or external services, has become more automated and efficient. By employing LLMs, organizations can generate annotated datasets quickly, facilitating machine learning model training and other analytical tasks.
The global augmented analytics market has witnessed significant growth, valued at $8.95 billion in 2023. Experts expect it to reach $11.66 billion in 2024 and surge to $91.46 billion by 2032.
4. Natural language processing (NLP)
In 2024, developing proprietary NLP models has become less common as more organizations prefer to leverage existing models via APIs. Building and maintaining custom NLP models is often seen as unnecessary, except for cases where there is a need to keep data internal due to privacy or security concerns.
Many complex tasks that were previously challenging, such as reference resolution and database matching, have become significantly easier with advanced models like ChatGPT. For example, merging databases from different clients with varying structures and field names is a task that traditional NLP struggled with. However, ChatGPT and similar models can handle these tasks more effectively, offering more accurate and efficient solutions.
These advancements are primarily beneficial for high-resource languages, where large models perform exceptionally well. However, their effectiveness diminishes for languages with fewer resources and less available training data. Moreover, while LLMs excel in many areas, code generation is still an emerging capability and currently performs less reliably. This remains an area of ongoing improvement.
The market size in the Natural Language Processing market is projected to reach US$36 billion in 2024. It is expected to show an annual growth rate of 27.55%, resulting in a market volume of US$156.80bn by 2030.
5. Data fabric
Data fabric is an emerging architectural approach that enables seamless data integration and management across diverse data environments. It provides a unified view of data by connecting disparate data sources, whether on-premises or in the cloud.
Previously, multimodal models required training multiple models for various types of data, such as graphics, sound, and text, and then combining their outputs. Now, the approach has shifted to integrating all these data types into a single, large contextual vector, which can then be processed collectively. This unified method allows for more efficient handling of diverse data sources. This technique not only simplifies the model architecture but also enhances the performance and accuracy of the analysis.
By the end of 2024, 25% of data management suppliers, up from 5% currently, will offer a complete foundation for data fabric.
6. Graph analytics
Graph analytics focuses on relationships between data points, making it particularly useful for analyzing complex networks such as social media connections, supply chains, and fraud detection.
Traditionally, graphs are defined using either adjacency matrices or adjacency lists (edge lists). Modern approaches involve converting these graph structures into vector representations. This process, often referred to as “flattening” the graph, enables neural networks to analyze the data more effectively.
Transformer-based models have become integral to this process. By encoding graph data into vector formats, transformers can process and interpret complex relationships within the graph. This method allows for sophisticated analysis and provides insightful answers to queries about the graph data.
7. Computer vision
Two key technologies driving changes in this field are transformers and diffusion models, which have revolutionized how data is generated and analyzed.
Transformers, originally designed for natural language processing, have found significant applications in computer vision. These models excel at capturing long-range dependencies and contextual relationships within data, making them ideal for complex image analysis tasks such as image classification, image segmentation, and object recognition.
Diffusion models have emerged as powerful tools for data generation in computer vision. These models work by iteratively refining random noise into coherent images, allowing for the creation of highly realistic and diverse datasets. They are used for creating synthetic data: large, high-quality datasets for training computer vision models. This is particularly useful in scenarios where collecting real-world data is challenging or expensive.
Moreover, these models enable the generation of images in various artistic styles, facilitating creative applications in design and media.
Conclusion
The landscape of data analytics is rapidly evolving, driven by advancements in technology and changing business needs. Staying abreast of these top trends is essential for organizations to enhance their efficiency and maintain a competitive edge in the market.
Read more: