Sora is a text-to-video free AI tool that allows creators to generate high-quality videos based on a text prompt. The model can be useful in SMM, cinematography, entertainment and anywhere else where quality video content is required.
In this article, you will learn what to expect from this new generation AI tool.
What is text-to-video AI?
An AI text-to-video model is a type of artificial intelligence that can generate videos based on textual descriptions or prompts provided by a user. This technology combines natural language processing (NLP) with computer vision techniques to understand and interpret text descriptions, then translate them into visual sequences.
Here’s how a text-to-video AI model typically works and what it can do:
- Text understanding. The AI model first processes and understands the textual input provided by the user. This can include descriptions of scenes, actions, objects, or scenarios that the user wants to see in the video.
- Scene generation. Based on the text input, the AI generates a sequence of images or frames that represent the described scene. It uses computer vision algorithms to visualize the elements mentioned in the text, such as objects, characters, environments, and actions. Some models generate them frame-by-frame, some, like Stable Diffusion, create a visual noise and gradually add details.
- Visual interpretation. The AI model interprets the text to determine how the visual elements should be arranged, animated, and presented in the video. This involves understanding spatial relationships, movements, camera angles, and other visual details. For example, if an object is moving, the AI needs to predict the trajectory of its movement.
- Video synthesis. Using generative techniques, AI combines the generated frames into a coherent video sequence. It can create animations, transitions, and effects to bring the described scene to life.
- Quality and realism. Advanced text-to-video AI models aim to produce high-quality and realistic videos that closely match the user’s description. They may incorporate deep learning, neural networks, and sophisticated algorithms to improve visual fidelity and realism.
- Creative applications. Text-to-video AI models have a wide range of applications, from content creation for storytelling, advertising, and entertainment to educational purposes, virtual simulations, and artistic expression. They enable users to visualize ideas, concepts, and narratives in a dynamic and engaging format.
- Limitations. While text-to-video AI models have made significant advancements, they still face challenges in accurately interpreting complex or abstract text descriptions, handling diverse visual scenarios, and achieving complete realism. Issues such as context understanding, scene composition, and nuanced motion representation remain areas of ongoing research and development.
What is Sora?
The first major developments in text-to-video generation happened in 2022. For the last few years, Meta and Google were independently working on models that could create short video clips based on text descriptions and/or static images. The quality of these videos was low (no more than 512×512 pixels), while the videos didn’t exceed 5 seconds.
Sora from OpenAI is a neural network capable of generating high-resolution videos based on text input. It can generate videos up to a minute in length while maintaining visualization quality.
Here is an example:
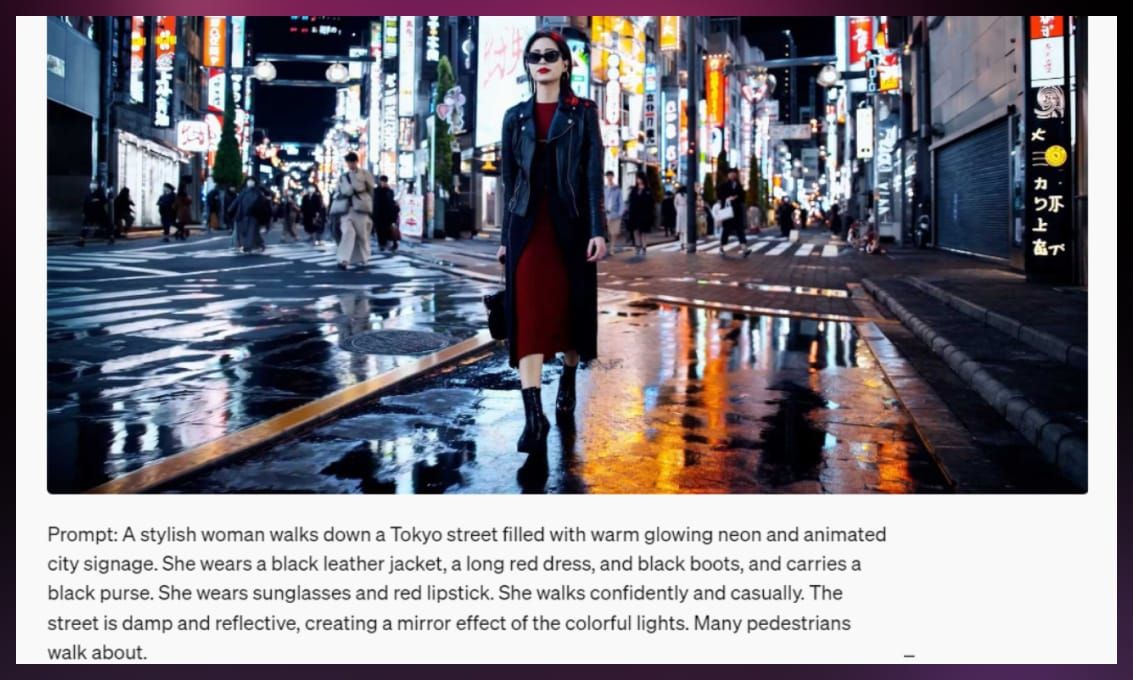
One of Sora’s key features is that it can generate complex scenes with multiple characters, specific types of movements, and precise details of objects and backgrounds.
However, the model also has its limitations. It might not understand specific cause-and-effect examples. For example, characters may appear holding objects out of nowhere. It can also mix up left and right or commit other minor mistakes.
For now, Sora is only available to a limited group of testers including selected visual artists, designers, and cinematographers to gather feedback and improve the platform before public release.
How does it work?
The underlying architecture of Sora combines diffusion and transformer models. Here’s a detailed breakdown of how Sora works:
- Diffusion architecture. Sora utilizes a diffusion-based approach similar to other neural networks like Stable Diffusion, and Midjourney. Instead of directly generating each frame, SORA starts with random noise and gradually transforms it into an image that matches the textual description provided as input.
- Transformer architecture. Sora also incorporates transformer models to handle spatial and temporal aspects of video generation. Transformers enable SORA to analyze both the spatial information (like the content of each frame) and temporal information (how the content changes over time) simultaneously.
- Text input processing. When a user inputs a textual description, Sora first processes this text. Unlike traditional models that use tokens, it employs patches as its input representation. These patches are generated based on the text prompt and serve as the initial data for video synthesis.
- Noise injection and prediction. Sora combines the processed text input with randomly generated noise. The network’s goal is to predict output patches that correspond to the description provided. Through multiple iterations, it refines these predictions to create more accurate representations of the desired video content.
- Training on text-to-video data. The neural network has been trained on a vast dataset containing pairs of text descriptions and corresponding videos. This extensive training allows Sora to understand the nuances of language and accurately translate them into visual data.
- Spatial-temporal patch analysis. After initial processing, the network breaks down the input data into spatial-temporal patches. These patches serve as the fundamental units for video synthesis, enabling Sora to focus on specific regions and time intervals within the generated content.
- Dynamic modeling for realistic motion. Sora employs dynamic modeling techniques to predict and visualize video motion realistically. This includes predicting how objects move and interact within the video, ensuring smooth transitions and lifelike animations.
- Resolution enhancement. The output starts with low-resolution latent representations, which are progressively refined to generate high-resolution video frames. This iterative process enhances the visual quality of the final video output.
What does Sora still need to improve?
Despite all its advanced technologies, Sora doesn’t always understand specific cause-and-effect relationships and may inaccurately model the physics of basic interactions. Moreover, the way objects behave in certain frames may appear unrealistic.
Conclusion
Sora is a state-of-the-art text-to-video generation model. It is able to generate high-quality videos and make them longer than competitor’s models from Meta and Google. However, it also has limitations that still need to be addressed, such as inconsistencies in object generation and unrealistic movement patterns. Today Sora is still being tested and isn’t available to the general public. However, upon release, it will most likely revolutionize video graphics and design as we know them.
If you want to learn more about AI and neural networks, read our other articles: