The market for AI in healthcare is expanding and is projected to grow in the coming years. This technology has already demonstrated significant capabilities in diagnostics and biomedical research. AI’s application in healthcare spans various areas, including early disease detection, personalized medicine, robotic surgeries, and patient management systems. Understanding its current state and potential future developments is crucial for healthcare professionals and policymakers.
In this blog post, we provide an overview of AI applications in healthcare, based on a webinar organized by the Instituto Universitario Piaget (Viseu) and presented by Serokell’s AI Team Lead, Ivan Smetannikov.
What are the general applications of AI in medicine?
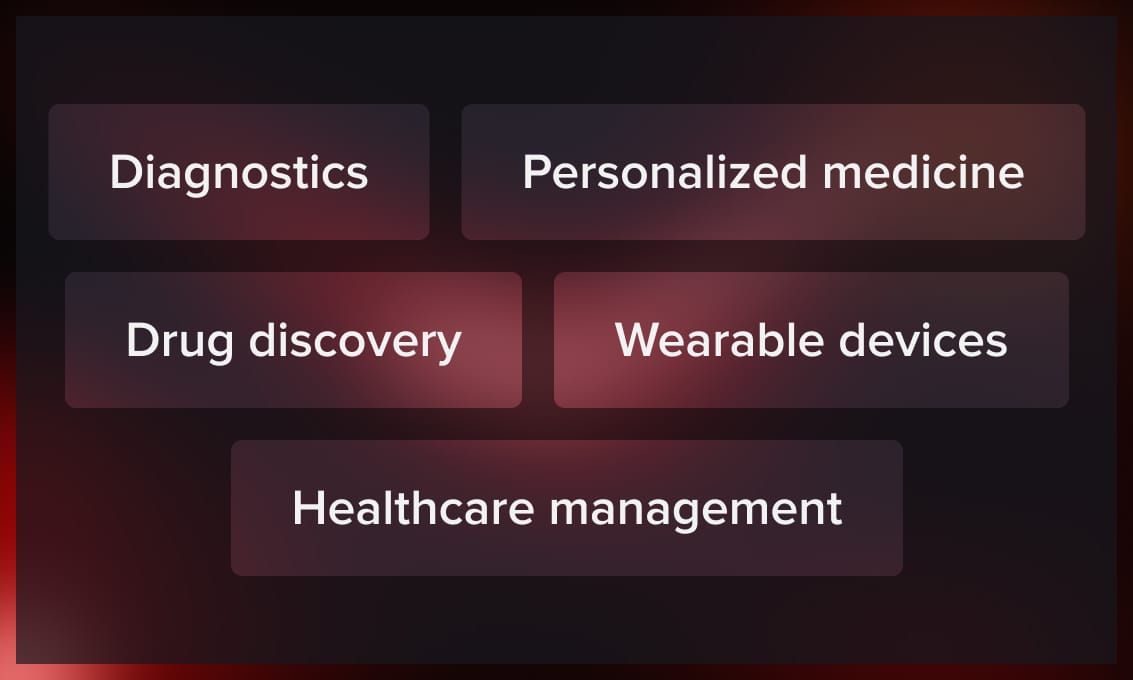
AI finds applications across all aspects of healthcare, from diagnostics and online consulting to treatment and healthcare management. By efficiently predicting patient outcomes and customizing treatments to individual needs, it holds promise in combating diseases such as cancer, Alzheimer’s, heart disease, and diabetes at early stages, as well as discovering cures for previously untreatable diseases. In other words, AI can potentially contribute to saving thousands or even millions of lives and enhancing the health of patients with chronic conditions.
Below are several examples of AI implementation in medtech:
Diagnostics
AI models trained on visual data from diseases at different stages can recognize the signals of such illnesses early.
Personalized medicine
AI analyzes patients’ genetic information and medical history to assist doctors in creating customized medications based on their unique genetic makeup, maximizing treatment effectiveness for individuals.
Drug discovery
AI algorithms calculate the probabilities of drug effectiveness, allowing the weakest methods to be dismissed from further investigation, thus accelerating the drug discovery process.
Wearable devices
AI-equipped devices continuously monitor vital signs, predicting potential health issues before they become serious. This is particularly important for managing conditions like diabetes and heart disease.
Healthcare management
Artificial intelligence automates routine tasks such as medical reporting and administrative duties, reducing bureaucratic workloads and streamlining healthcare management.
What ML methods are used in medicine?
Although many advanced AI/ML models are used in other industries, the list is limited for medtech and healthcare. In this field, we only use methods that can guarantee the highest level of validity (probability) and transparent results interpretation.
To perform analytical and predictive tasks, three main types of machine learning analysis are used:
- Classification: For example, it can be used to sort screening data into homogeneous categories—with or without anomalies anomalies—for diagnosing diseases.
- Regression: Predicts values, such as forecasting available hospital beds based on the average treatment lengths of patients and their treatment prescriptions.
- Clustering: Groups similar data points. This is helpful in patient segmentation and the development of personalized treatment plans.
Those three types of analysis are applied to the following tasks:
- Anomaly detection: Identifies unusual data points, such as atypical patient vital signs or a complex set of symptoms.
- Recommendation systems: Suggest relevant items to users. They recommend treatment options, lifestyle changes, or preventive measures based on a patient’s health history and current condition.
- Active learning: Enhances diagnostic models by selecting the most informative samples for human experts to label, improving model accuracy.
- Natural Language Processing (NLP): Deals with interactions between humans and natural language algorithms. It is used in healthcare for extracting information from clinical notes and patient records, and facilitating communication through chatbots and virtual assistants.
- Time series analysis: Predicts trends based on monitoring patients’ conditions. This is essential for forecasting various health-related problems.
- Reinforcement learning: Involves AI agents making decisions to maximize rewards. Optimizes treatment protocols personalized for patient care based on feedback and behavior.
- Dimensionality reduction: Simplifies the analysis of large datasets by retaining only essential information. It Improves interpretability of results and aids in the search for new compounds.
Generative AI
All the tasks described above are performed by the most common form of artificial intelligence today, generative AI.
Generative AI (GenAI) refers to artificial intelligence systems that can create medical reports, recognize diagnostic images, or recommend treatment plans based on learning patterns from existing healthcare data. These systems use advanced models like neural networks to generate outputs.
To enhance the efficiency of generative AI, traditional algorithms can be incorporated into these systems to solve specific sub-problems or optimize certain components in healthcare applications.
- Hidden Markov Models (HMMs): Used for sequence prediction and generation, particularly in speech and language modeling.
- Gaussian Mixture Models (GMMs): Employed for clustering, density estimation, and as components in more complex generative models like variational autoencoders.
- Bayesian Networks: Provide a framework for reasoning under uncertainty, useful in generative AI for creating models that capture dependencies between variables. Read more about Bayesian networks in our blog post.
- Autoregressive models: Utilized to model and generate time series data.
- Markov Chain Monte Carlo (MCMC) methods: Applied for sampling from complex, high-dimensional distributions, which is essential in training and inference of probabilistic models.
- Latent Dirichlet Allocation (LDA): Used for topic modeling, which can help in generating contextually relevant text and understanding document structures.
- Genetic Algorithms and Evolutionary Computation optimization methods: Employedfor optimizing neural network architectures and hyperparameters, as well as evolving creative solutions and designs.
Limitations of GenAI
When we speak of generative AI in healthcare, we most often refer to Large Language Models (LLMs), the most well-known example of which is ChatGPT.
Large Language Models (LLMs) operate by predicting the next word in a sequence, using the context of the preceding dialogue. This has several limitations.
- The fundamental limitation of LLMs lies in their nature as** probabilistic models**. They generate responses by selecting the most statistically likely words based on their training data, rather than through genuine comprehension of the question or subject matter.
- LLMs struggle to retain information beyond their context window. The transformer architecture, which underlies most LLMs, inherently loses context over time. This is particularly problematic with large datasets, such as comprehensive medical records. Consequences include:
- Degraded prediction quality for information outside the context window
- Potential for “hallucinations” or fabricated information when the model lacks necessary context.
- Another limitation to be aware of is the “world model” of LLMs: their accumulated knowledge is reconstructed from texts, not from real-world experiences, leading to potential errors.
- In the healthcare sector, LLMs can be provided as** external APIs** (e.g., OpenAI), raising concerns about data privacy and security, or run locally within an organization to maintain control over sensitive data.
To address all these concerns and overcome the limitations, more AI research is needed. That’s why investments in this project are vital.
AI in healthcare market overview
According to Statista, the global AI in healthcare market was worth around 11 billion U.S. dollars in 2021. It is forecasted to reach almost $188 billion by 2030, with a compound annual growth rate of 37 percent from 2022 to 2030.
Fortune Business Insights expects the market to grow from $27.69 billion in 2024 to $490.96 billion by 2032.
Binariks provides similar estimates, reporting the market size at $16.3 billion in 2022 and projecting it to reach $173.55 billion by 2029.
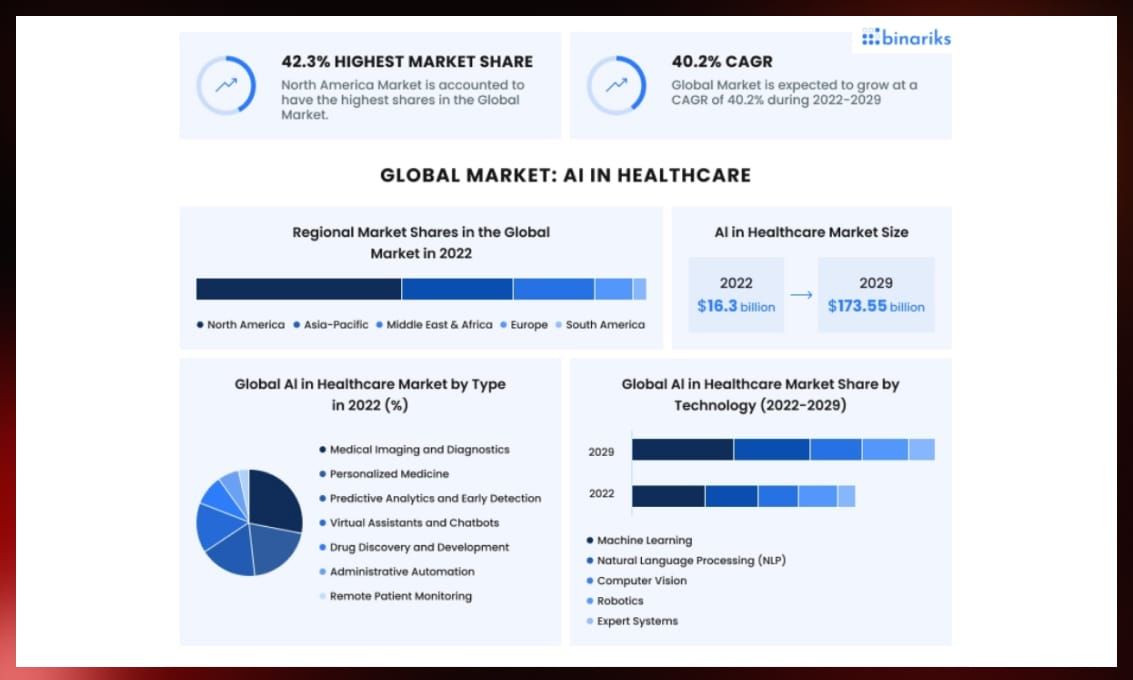
How is AI used for drug discovery?
Now that we have covered the working principles of AI, let’s explore how they are applied in one specific healthcare field—drug discovery.
The traditional process of bringing a new drug to market is highly time-consuming and expensive, often taking 10 to 15 years and costing up to $1 billion, with high failure rates, especially during clinical trials. AI significantly increases efficiency, reduces costs, and decreases failure rates at various stages. Below, we explain with a real example how artificial intelligence aids in each stage of the drug discovery process.
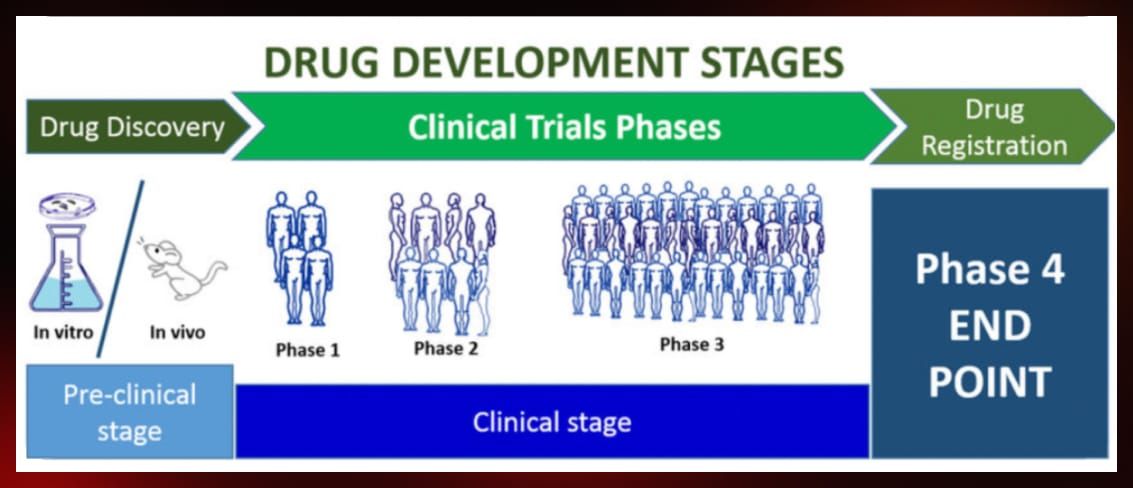
Target identification and validation
The first stage is target identification and validation, which involves identifying a biological target, usually a protein, implicated in a disease, and validating its role.
AI helps in druggability assessment by exploring the properties of proteins and assessing their stability for future compound screens. It analyzes vast amounts of biological and genetic data to identify potential disease mechanisms and drug targets, significantly reducing the search time and improving the overall efficiency.
Hit discovery
The next stage is hit discovery, a broad search for potential compounds with the desired biological activity, using high-throughput screening and large compound libraries. The goal is to generate a vast number of compounds, though only a small fraction will show activity. AI streamlines the hit discovery phase by predicting the activity of chemical compounds against specific biological targets.
Lead optimization
In the lead optimization phase, potential drug candidates are determined, and their properties are improved. AI is used to predict ADME profiles (absorption, distribution, metabolism, excretion, and toxicity), forecast the effects of molecular modifications, and model compound properties, reducing the need for extensive physical synthesis and testing.
Moreover, AI can analyze complex structure-activity relationship data, suggesting modifications to enhance compound efficacy and reduce toxicity. It also helps predict and mitigate unforeseen safety issues and identify biomarkers for drug response.
Preclinical testing
The next stage is preclinical testing, which consists in evaluating selected and optimized drug candidates in vitro (test tubes) and in vivo (on animals) to validate their safety and efficacy. AI models predict how new drugs will behave in humans.
Clinical trials
AI helps design clinical trials in the optimal way by predicting various side effects, dosing, and efficacy for different groups. This reduces the size of test groups and helps in selecting underrepresented patients for trials.
Regulatory approval
The regulatory approval stage, handled by the FDA in the US, requires proof that the medication can be marketed. AI can analyze large volumes of data to quantify outcomes, ensure quality control, and detect outliers, inconsistencies, and missing values. It also automates the generation of submission documents, speeding up the approval process.
Post-market surveillance
Post-market surveillance involves observing medical products on the market to understand their performance in a broader population. AI plays a crucial role in this process by monitoring real-time data from wearable devices and patient registries to detect adverse events and analyze patient feedback. It can also pinpoint higher-risk patients, enabling targeted monitoring. Additionally, automated reporting can be used for compliance checks to ensure safety protocols are updated promptly and stakeholders are informed of regulatory changes.
AI-powered drug discovery use cases
Now, let’s examine some of the most interesting and promising use cases of AI in drug discovery.
Prediction of binding affinity: AtomNet
AtomNet is a deep learning application that uses 3D convolutional neural networks to predict the binding affinity of small molecules to proteins. It captures detailed information about binding pockets and interactions and identifies high-affinity molecules from virtual screening libraries. It can also assist in the lead optimization step by predicting how specific chemical modifications might affect binding affinity, as well as in drug repurposing.
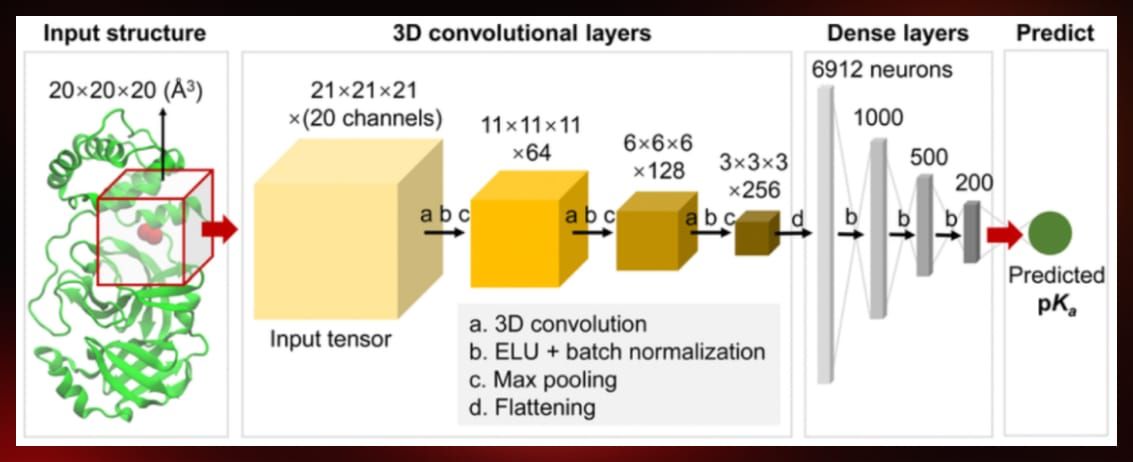
Drug candidates identification: Insilico Medicine
Insilico Medicine is an AI-driven platform that generates new molecular structures and evaluates their potential as drug candidates. It employs generative adversarial networks and reinforcement learning to identify promising drug candidates much faster than traditional methods. Reports indicate it can go from initial stages to testing in less than 46 days, whereas the traditional process may take years.
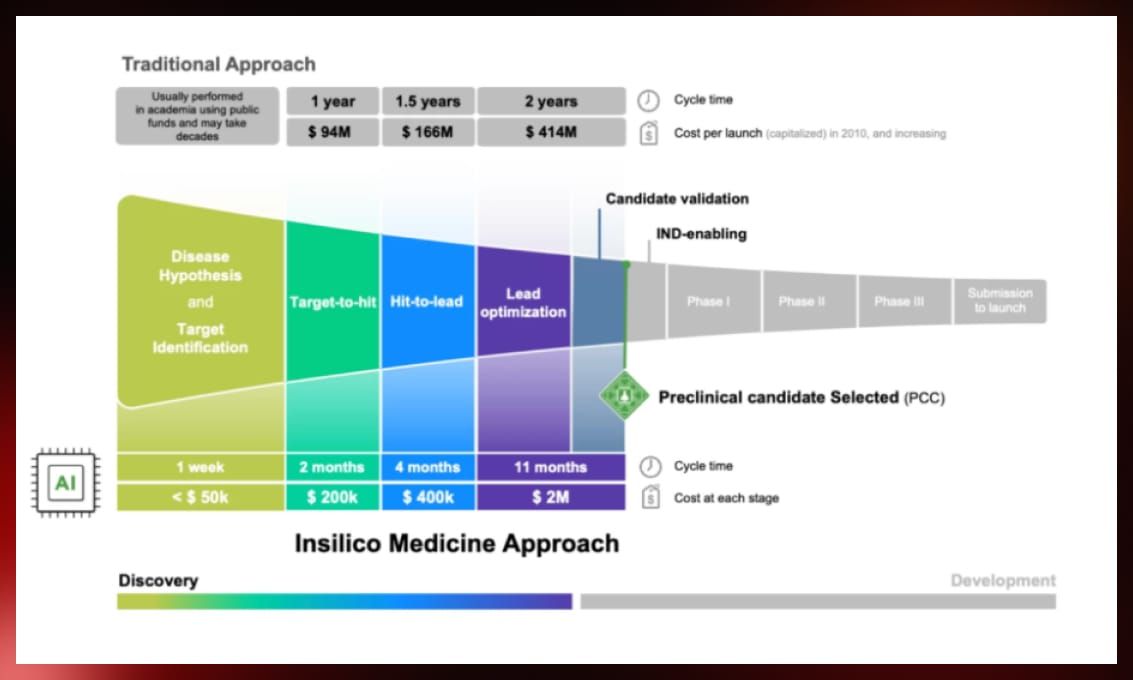
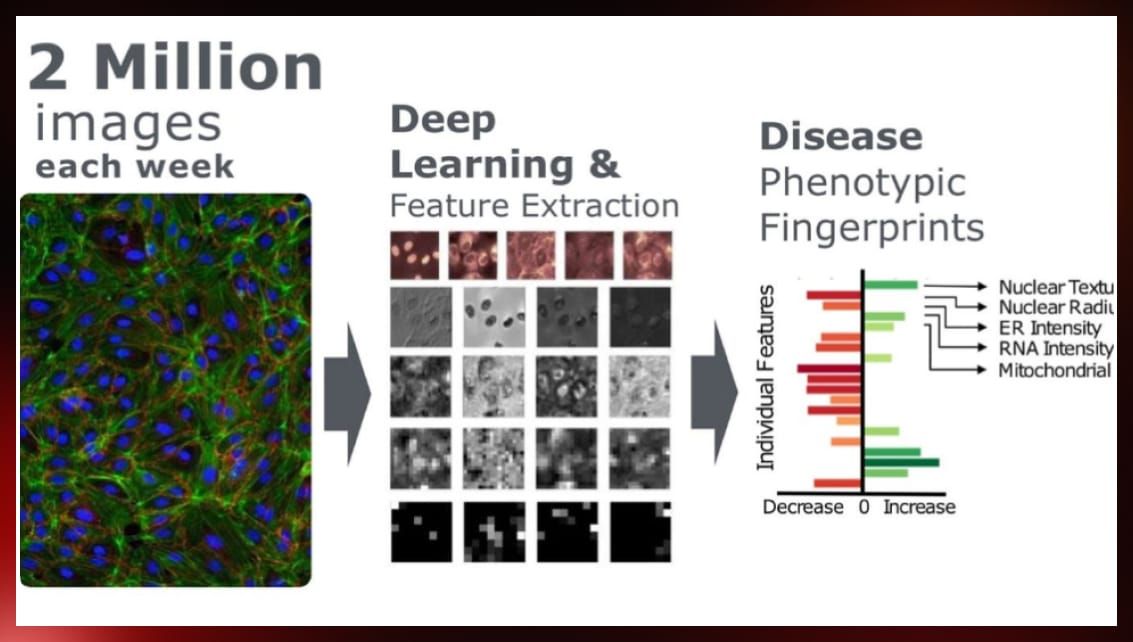
Compounds testing: Recursion
Recursion combines AI with a high-throughput screening system to map cellular biology and identify potential treatments. Their platform can test thousands of compounds across multiple biological contexts simultaneously, using automated microscope image analysis and screening. Computer vision algorithms detect changes in cellular phenotypes, identify patterns, and predict potential treatments.
Protein structure prediction: Alpha Fold
AlphaFold is a protein structure database developed by Google DeepMind and EMBL-EBI (European Bioinformatics Institute). It allows researchers to predict the 3D structures of proteins with high accuracy using a transformer model.
The machine learning model has two main components: one predicts the distance and angles between pairs of amino acids using an attention-based network, and the other focuses on relevant parts of amino acid sequences to predict the structured cell. Its predictions have been verified through methods like X-ray crystallography and have been significant in the field, especially for generating new proteins and drugs.
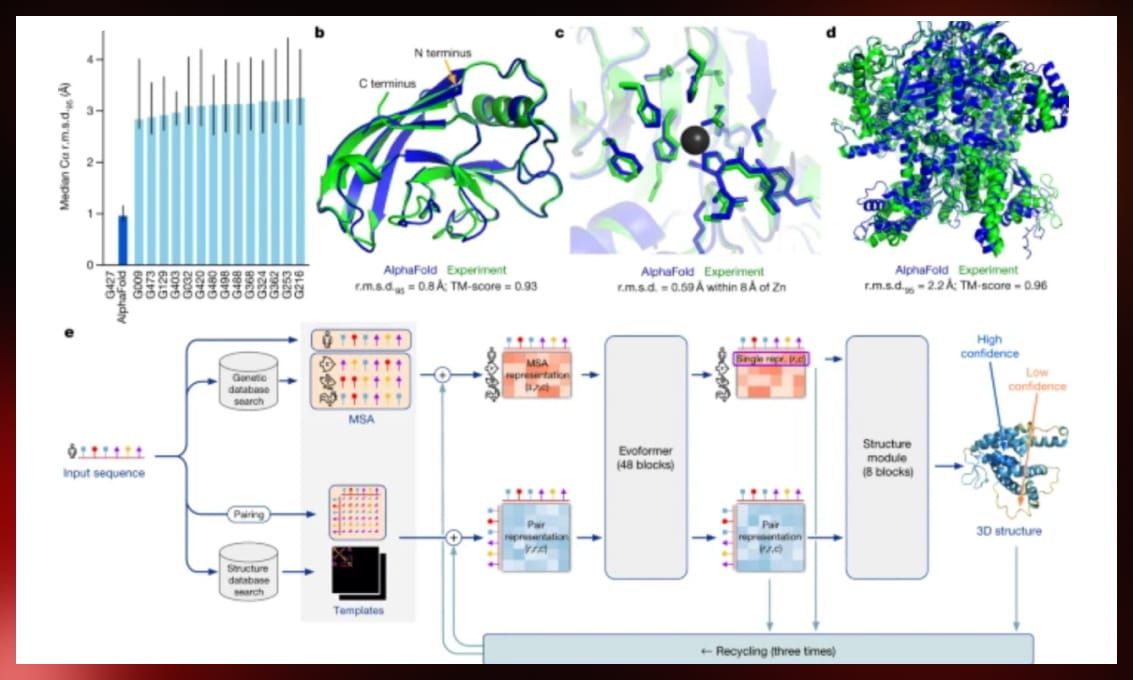
Halicin repurposing: MIT
Researchers at MIT used graph neural networks to analyze hundreds of millions of chemical compounds in just three days, discovering that halicin, initially used for diabetes treatment, is effective against antibiotic-resistant strains.
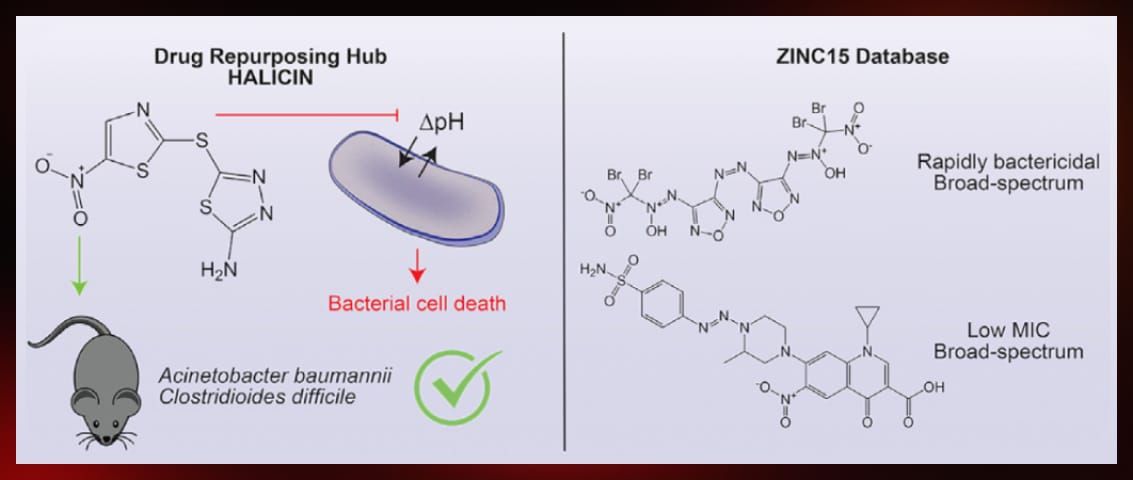
Serokell’s work in AI research in medicine
Serokell has extensive experience in the healthcare sector, particularly in virology. We collaborated with a major pharmaceutical company to develop models for automated feature selection, specifically addressing the problem of dementia risk reduction for cancer drug synthesis. We created an algorithm that selects the most relevant genes from tens of thousands. Our solution significantly improved efficiency by reducing the time required for model building and result generation from several weeks to just a few days. It also eliminated the need for expensive GPU servers, making the process more cost-effective by utilizing CPUs instead.
Additionally, our ML team worked on drug repurposing projects with Elsevier, employing graph neural networks to predict interactions between small molecules and diseases. Elsevier provided a dataset of thousands of research papers condensed into a graph format. Our application, powered by graph neural networks, analyzed relationships within the dataset, matching papers related to diseases, proteins, and small molecules, and identifying missing edges—a common problem in graph analysis.
AI safety in healthcare
The major safety concerns for AI use in healthcare include transparency, bias, clinical validation, regulatory compliance, and data privacy.
Transparency
The “black box” nature of deep learning models necessitates additional measures for their use in healthcare. Two main approaches are possible here:
- Developing AI systems that explain their decisions in understandable terms,
- Creating low-accuracy, high-precision systems that can be validated by humans.
Bias
Ensuring that datasets are diverse and representative is crucial, as AI performs better with well-represented data, reducing the risk of disparities in treatment and diagnosis across different patient populations.
Clinical validation
Clinical validation is also necessary before deploying AI tools in real-world scenarios. This involves conducting rigorous trials and studies to assess their performance, accuracy, and reliability in clinical settings.
Regulatory compliance and data privacy
Given the sensitivity of patient data, robust systems are needed to prevent hacking and data leakage. Compliance with regulations such as HIPAA in the United States and GDPR in Europe is essential to ensure that patient data is handled securely and ethically. This involves implementing strong encryption methods, secure data storage solutions, and regular audits to identify and mitigate potential vulnerabilities. Additionally, ensuring transparency in data usage and obtaining proper patient consent are critical components in maintaining trust and compliance with legal standards.
Conclusion
The integration of AI in healthcare offers a transformative opportunity to enhance patient care and streamline medical and drug discovery processes. However, the importance of human-AI collaboration cannot be overstated. That is why AI should serve as an augmentative tool rather than a replacement for medical experts.
As AI continues to evolve, the education and training of medical professionals in these technologies is becoming increasingly important.